The remarkable evolution of Artificial Intelligence (AI) systems represents a paradigm shift in the relationship between humans and machines. This transformation is evident in the seamless interactions facilitated by these advanced systems, where adaptability emerges as a defining characteristic, resonating with the fundamental human capacity to learn from experience and predict behaviour.
AI mimics human learning
One facet of AI that aligns closely with human cognitive processes is Reinforcement Learning (RL). RL mimics the human learning paradigm by allowing AI systems to learn through interaction with an environment, receiving feedback in the form of rewards or penalties. By contrast, Large Language Models (LLMs) play a crucial role in pattern recognition, capturing the intricate nuances of human language and behaviour. These models, such as ChatGPT and BERT, excel in understanding contextual information, grasping the subtleties of language, and predicting user intent. Leveraging vast datasets, LLMs acquire a comprehensive understanding of linguistic patterns, enabling them to generate human-like responses and adapt to some of the user behaviour, sometimes with remarkable accuracy.
The synergy between RL and LLMs creates a powerful predictor of human behaviour. RL contributes the ability to learn from interactions and adapt, while LLMs enhance the prediction capabilities through pattern recognition. AI systems based on RL can thus display a form of behavioural synchrony. At its core, RL enables AI systems to learn optimal sequences of actions in interactive environments to achieve a policy. Analogous to a child touching a hot surface and learning to avoid it, these AI systems adapt based on the positive or negative feedback they receive.
AI replicates human interactions
AI agents using deep reinforcement learning, such as Google DeepMind’s AlphaZero, learn and improve by playing millions of games against themselves, thereby refining their strategies over time. This self-improvement process in AI involves an agent iteratively learning from its own actions and outcomes. Similarly, in human interactions, brain synchrony occurs when individuals engage in cooperative tasks, leading to aligned patterns of brain activity that facilitate shared understanding and collaboration. Unlike AI, humans achieve this synchrony through interaction with others rather than themselves.
What’s more, AI systems can also learn from interactions with humans. Just as human brain synchrony enhances cooperation and understanding, AI systems can improve and align their responses through extensive iterative learning from human interactions. While AI systems do not literally share knowledge as human brains do, they become repositories of data inherited from these interactions, which corresponds to a form of knowledge. This process of learning from vast datasets, including human interactions, can be seen as a form of ‘collective memory’. This analogy highlights the potential for AI systems to evolve while being influenced by humans, while also influencing humans through their use, indicating a form of ‘computational synchrony’ that could be seen as an analogue to human brain synchrony.
In addition, AI systems enabled with social cue recognition are being designed to detect and respond to human emotions. These ‘Affective Computing’ systems, as coined by Rosalind Picard in 19951, can interpret human facial expressions, voice modulations, and even text to gauge emotions and then respond accordingly. An AI assistant that can detect user frustration in real-time and adjust its responses or assistance strategy is a rudimentary form of behavioural synchronisation based on immediate feedback.
For instance, affective computing encompasses technologies like emotion recognition software that analyses facial expressions and voice tone to determine a person’s emotional state. Real-time sentiment analysis in text and voice allows AI to adjust its interactions to be more empathetic and effective. This capability is increasingly used in customer service chatbots and virtual assistants to improve user experience by making interactions feel more natural and responsive.
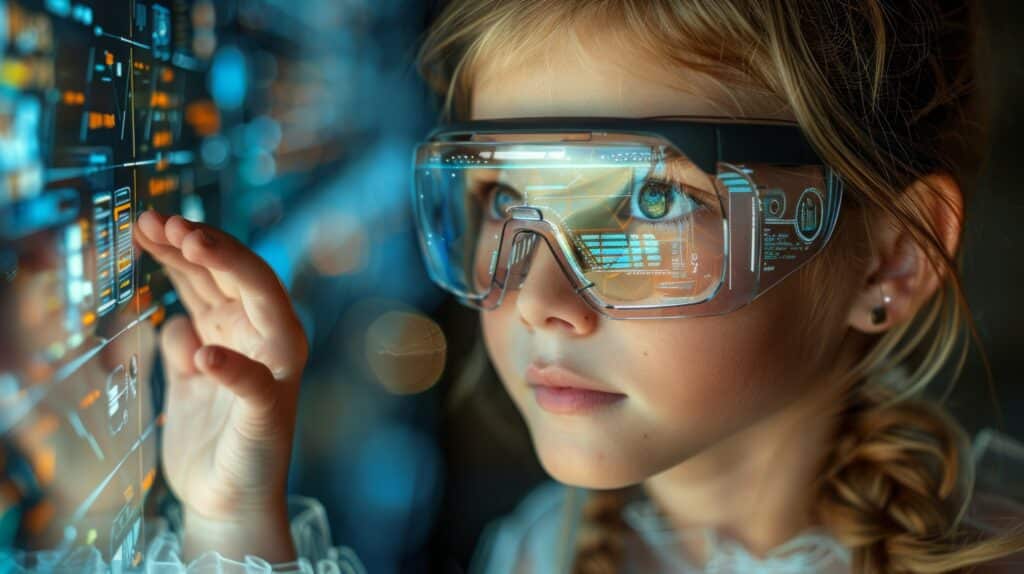
Just as humans adjust their behaviour in response to social cues, adaptive AI systems modify their actions based on user input, potentially leading to a form of ‘synchronisation’ over time. Assessing the social competence of such an AI system could be done by adapting tools like the Social Responsiveness Scale (SRS)—a well-validated psychiatric instrument that measures how adept an individual is at modifying their behaviour to fit the behaviour and disposition of a social partner, a proxy for ‘theory of mind,’ which refers to the ability to attribute mental states—such as beliefs, intents, desires, emotions, and knowledge—to oneself and to others.
Moving towards resonance
Brain-Computer Interfaces (BCIs) have ushered in a transformative era in which thoughts can be translated into digital commands and human communication. Companies like Neuralink are making strides developing interfaces that enable paralysed individuals to control devices directly with their thoughts. Connecting direct recordings of brain activity with AI systems, researchers enabled an individual to speak at normal conversational speed after being mute for more than a decade following a stroke. AI systems can also be used to decode not only what an individual is reading but what they are thinking based on non-invasive measures of brain activity using functional MRI.
Based on these advances, it’s not far-fetched to imagine a future scenario in which a professional uses a non-invasive BCI (e.g., wearable brainwave monitors such as Cogwear, Emotiv, or Muse) to communicate with AI design software. The software, recognising the designer’s neural patterns associated with creativity or dissatisfaction, could instantaneously adjust its design proposals, achieving a level of synchrony previously thought to be the realm of science fiction. This technological frontier holds the promise of a distinctive form of synchrony, where the interplay between the human brain and AI transcends mere command interpretation, opening up a future in which AI resonates with human thoughts and emotions.
Crucially, the resonance envisioned here transcends the behavioural domain to encompass communication as well. As BCIs evolve, the potential for outward expressions becomes pivotal. Beyond mere command execution, the integration of facial cues, tone of voice, and other non-verbal cues into AI’s responses amplifies the channels for resonance. This expansion into multimodal communication may enrich synchrony by capturing elements from the holistic nature of human expression, creating a more immersive and natural interaction.
However, the concept of resonance also presents the challenge of navigating the uncanny valley, a phenomenon where humanoid entities that closely resemble humans provoke discomfort. Striking the right balance is paramount, ensuring the AI’s responsiveness aligns authentically with human expressions, without entering the discomfiting realm of the uncanny valley. The potential of BCIs to foster synchrony between the human brain and AI introduces promising yet challenging prospects for human-computer collaboration.
Turning to neuroscience
Neuroscience not only illuminates the basis of biological intelligence but may also guide development of artificial intelligence2. Considering evolutionary constraints like space and communication efficiency, which have shaped the emergence of efficient systems in nature, prompts exploration of embedding similar constraints in AI systems, envisioning organically evolving artificial environments optimised for efficiency and environmental sustainability, the focus of research in so-called “neuromorphic computing.”
For example, oscillatory neural activity appears to boost communication between distant brain areas. The brain employs a theta-gamma rhythm to package and transmit information, similar to a postal service, thereby enhancing efficient data transmission and retrieval3. This interplay has been likened to an advanced data transmission system, where low-frequency alpha and beta brain waves suppress neural activity associated with predictable stimuli, allowing neurons in sensory regions to highlight unexpected stimuli via higher-frequency gamma waves. Bastos et al.4 found that inhibitory predictions carried by alpha/beta waves typically flow backward through deeper cortical layers, while excitatory gamma waves conveying information about novel stimuli propagate forward through superficial layers.
Recent AI experiments, particularly those involving OpenAI’s GPT‑4, unveil intriguing parallels with evolutionary learning.
In the mammalian brain, sharp wave ripples (SPW-Rs) exert widespread excitatory influence throughout the cortex and multiple subcortical nuclei5. Within these SPW-Rs, neuronal spiking is meticulously orchestrated both temporally and spatially by interneurons, facilitating the condensed reactivation of segments from waking neuronal sequences6. This orchestrated activity aids in the transmission of compressed hippocampal representations to distributed circuits, thereby reinforcing the process of memory consolidation7.
Recent AI experiments, particularly those involving OpenAI’s GPT‑4, unveil intriguing parallels with evolutionary learning. Unlike traditional task-oriented training, GPT‑4 learns from extensive datasets, refining its responses based on the accumulated ‘experiences’ – furthermore pattern recognition by GPTs parallels pattern recognition by layers of neurons in the brain. This approach mirrors the adaptability observed in natural evolution, where organisms refine their behaviours over time to better resonate with their environment.
From Brain Waves to AI Frequencies
Drawing inspiration from the architecture of the brain, neural networks in AI are constructed with nodes organised in layers that respond to inputs and then generate outputs. In the realm of human neural synchrony research, investigating the role of oscillations has proven to be a pivotal area of interest. High-frequency oscillatory neural activity stands out as a crucial element, demonstrating its ability to facilitate communication between distant brain areas. A particularly intriguing phenomenon in this context is the theta-gamma neural code, showcasing how our brains employ a distinctive method of ‘packaging’ and ‘transmitting’ information, reminiscent of a postal service meticulously wrapping packages for efficient delivery. This neural ‘packaging’ system orchestrates specific rhythms, akin to a coordinated dance, ensuring the streamlined transmission of information, and it is encapsulated in what is known as the theta-gamma rhythm.
This perspective aligns with the concept of “neuromorphic computing,” where AI architecture is based on neural circuitry. The key advantage of neuromorphic computing lies in its computational efficiency, addressing the significant energy consumption challenges faced by traditional AI models. The training of large AI models, such as those used in natural language processing or image recognition, can consume an exorbitant amount of energy. For instance, training a single AI model can emit as much carbon dioxide as five cars over their entire lifespan8. Moreover, researchers at the University of Massachusetts, Amherst, found that the carbon footprint of training deep learning models has been doubling approximately every 3.5 months, far outpacing improvements in computational efficiency9.
Neuromorphic computing offers a promising alternative. By mimicking the architecture of the human brain, neuromorphic systems aim to achieve higher computational efficiency and lower energy consumption compared to conventional AI architectures10. For example, IBM’s TrueNorth neuromorphic chip has demonstrated significant orders of magnitude in energy efficiency compared to traditional CPUs and GPUs11. Additionally, neuromorphic computing architectures are inherently suited for low-power, real-time processing tasks, making them ideal for applications like edge computing and autonomous systems, further contributing to energy savings and environmental sustainability.
Implications for society
In the realm of training and skill development, synchronised AI has the potential to personalise learning experiences based on an employee’s unique learning curve, facilitating faster and more effective skill acquisition. From a customer engagement standpoint, synchronised AI interfaces might more precisely understand and, in some cases, anticipate user expectations based on advanced behavioural patterns.
For operational efficiency, especially in sectors like manufacturing or logistics, AI systems working in coordination with each other can optimise processes, reduce waste, and strengthen the supply chain. This would lead to increased profitability, with an ever-met greater ability for sustainability considerations integrated. In risk management, synchronised AI systems analysing vast datasets collaboratively might better predict potential risks or market downturns, equipping businesses and other organisations to prepare or pivot before a crisis emerges to limit all related social and societal impact. Likewise, synchronised AI systems could provide insights for more efficient urban planning and environmental protection strategies. This could lead to better traffic management, energy conservation, and pollution control, enhancing the quality of life in urban areas.
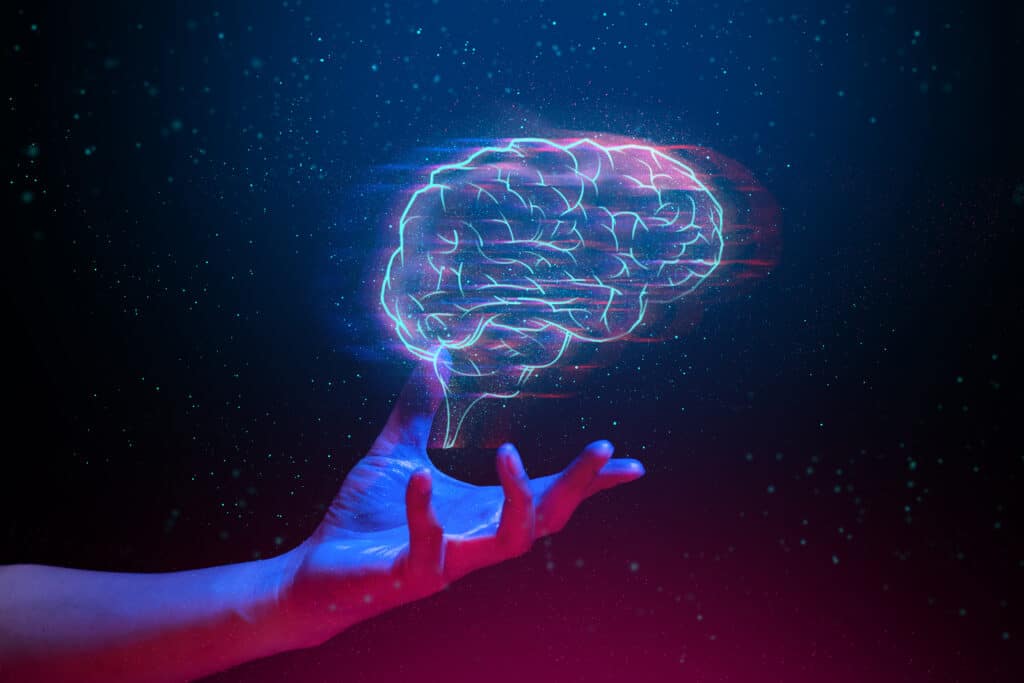
In various domains beyond business, deployment of AI with a prosocial orientation holds immense potential for the well-being of humanity and the planet. Particularly in healthcare, synchronisation between the human brain and AI systems could usher in a revolutionary era for patient care and medical research. Recent studies highlight the positive impact of clinicians synchronising their movements with patients, thereby increasing trust, and reducing pain. Extending this concept to AI chatbots or AI-enabled robotic caregivers that are synchronised with those under their ‘care’ holds the promise of enhancing patient experience and improving outcomes, as evidenced by recent research indicating that LLMs outperformed physicians in diagnosing illnesses, and patients preferred their interaction.
In the educational domain, the integration of AI systems with a focus on synchrony is equally promising. Research demonstrated that synchronized brain waves in high school classrooms were predictive of higher performance and happiness among students12. This study underscores the significance of neural synchrony in the learning environment. By leveraging AI tutoring systems capable of detecting and responding to students’ cognitive states in real-time, education technology can potentially replicate the positive outcomes observed in synchronised classroom settings. Incorporation of AI systems that resonate with students’ brain states has the potential to create a more conducive and effective learning atmosphere, optimizing engagement and fostering positive learning outcomes.
Perspectives and Potential
The excitement surrounding the prospects of brain-to-machine and machine-to-machine synchrony brings with it a set of paramount concerns that necessitate scrutiny and that are all but technical. Data privacy emerges as a critical apprehension, given the intimate nature of neural information being processed by these systems. The ethical dimensions of such synchronisation, particularly in the realm of AI decision-making, present complex challenges that require careful consideration1314.
Expanding on these concerns, two overarching issues demand heightened attention. Firstly, the preservation of human autonomy stands as a foundational principle. As we delve into the era of brain-machine synchrony, it becomes imperative to ensure that individuals retain their ability to make informed choices. Avoiding scenarios where individuals feel coerced or manipulated by technology is crucial in upholding ethical standards.
Secondly, the question of equity in access to these technologies emerges as a pressing matter. Currently, such advanced technologies are often costly and may not be accessible to all segments of society. This raises concerns about exacerbating existing inequalities15. A scenario where only certain privileged groups can harness the benefits of brain-machine synchrony might deepen societal divides. Moreover, the lack of awareness about these technologies further compounds issues of equitable access16.
The integration of AI with human cognition marks the threshold of an unprecedented era, where machines not only replicate human intelligence but also mirror intricate behavioural patterns and emotions. The potential synchronisation of AI with human intent and emotion holds the promise of redefining the nature of human-machine collaboration and, perhaps, even the essence of the human condition. The outcome of harmonising humans and machines will significantly impact humanity and the planet, contingent upon the guiding human aspirations in this pursuit, and open opportunities for an advanced human-centered AI experience, in a “Fusion Mode”, as coined in the “Artificial Integrity” concept. This raises a timeless question, reverberating through the course of human history: what do we value, and why?
A crucial point to emphasise is that the implications of synchronising humans and machines extend far beyond the realm of AI experts; it encompasses every individual. This underscores the necessity to raise awareness and engage the public at every stage of this transformative journey. As the development of AI progresses, it is essential to ensure that the ethical, societal, and existential dimensions are shaped by collective values and reflections, avoiding unilateral decisions by Big Tech that may not align with the broader interests of humanity. What happens next shapes our individual and collective future. Getting it right is our shared responsibility.