Quantum computing and AI: less compatible than expected?
- There is a belief that quantum computing could revolutionise artificial intelligence and in particular deep learning.
- However, quantum computing will not necessarily advance AI because it encounters difficulties in processing information from neural networks and voluminous data.
- In particular, quantum computers are very slow and only very short calculations can be carried out without breakdowns.
- However, AI machine learning is an essential tool for learning how to design and operate quantum computers today.
With a number of tech firms promising to be able to solve some small real-world problems within the next few years, it would seem that the world is on the cusp of a quantum computing breakthrough. As such there had been much hope that access to such quantum computing would transform artificial intelligence too. But a growing consensus suggests this may not yet be within reach.
What can be said about the origins behind the belief that quantum computing could revolutionise AI?
Filippo Vicentini. AI is a very wide-ranging term. So, I’ll focus on “deep learning”, which is behind the new technologies like text, audio and video generative models that we are seeing explode today. The idea that quantum computing could boost AI development became more prominent around 2018–19. Companies were coming out with early quantum computers with 1, 2, 3, or 4 noisy qubits. Because of their limitations, these machines could not be used to do larger real-world calculations, which is where we expect quantum computing to really shine. Instead, they were tasked with doing many short “quantum” subroutines (commonly known as quantum circuits), feeding back into a classical optimisation algorithm. This approach is strikingly similar to how neural networks are trained in deep learning.
The hope, back around that time, was that a reasonably sized “quantum circuit” would be more expressive — meaning it could present more complex solutions to a problem with fewer resources — than a neural network, thanks to quantum phenomena like interference and superposition. In short, this could mean that quantum circuits could enable algorithms that could learn to find correlations within data more effectively. Hence, the field of quantum machine learning was born, and several researchers started to try to bring ideas from one side to the other. There was a lot of excitement at the time.
Several companies have touted the advent of more powerful quantum computers in the next few years. Does that mean we should expect a leap forward in AI as well?
The brief answer would be no, I don’t think quantum computing will boost AI forward. It’s becoming increasingly clear that quantum computers will be very useful for applications that require limited input and output but a huge amount of processing power. For instance, to solve complex physics problems related to superconductivity or simulate chemical molecules. However, for anything related to big data and neural networks, the consensus is growing that it may ultimately not be worth the effort.
This position was recently laid out in a paper1 by Swiss National Supercomputing Centre’s Torsten Hoefler, Amazon’s Thomas Häner, and Microsoft’s Matthias Troyer. I just finished reviewing submissions for the QTML24 (quantum techniques in machine learning) conference and the tone of the quantum machine learning community was on a downward trend.
Why is that?
More and more experts are recognising that quantum computers will likely remain very slow when it comes to input and output of data. To give you an idea, we expect that a quantum computer that could exist maybe five years from now — if we are being optimistic — will have the same speed to read and write as an average computer from 1999 or 2000.
If we try to run quantum computers faster to increase the amount of data we can inject, we will start to introduce more errors in the calculation, and the result will deteriorate. There seems to be a speed limit for the operation of these machines above which the noise and errors are too strong to be corrected, even when we look about 20 years in the future.
Both classical and quantum computers are noisy. For one, a bit or a qubit, at some point, can randomly switch to a 1. While we can address this effectively in classical computers, we don’t have that technology in quantum computers. We estimate it will take at least another 15 years to develop fully fault-tolerant quantum computers. That means we can only do very ‘short’ calculations.
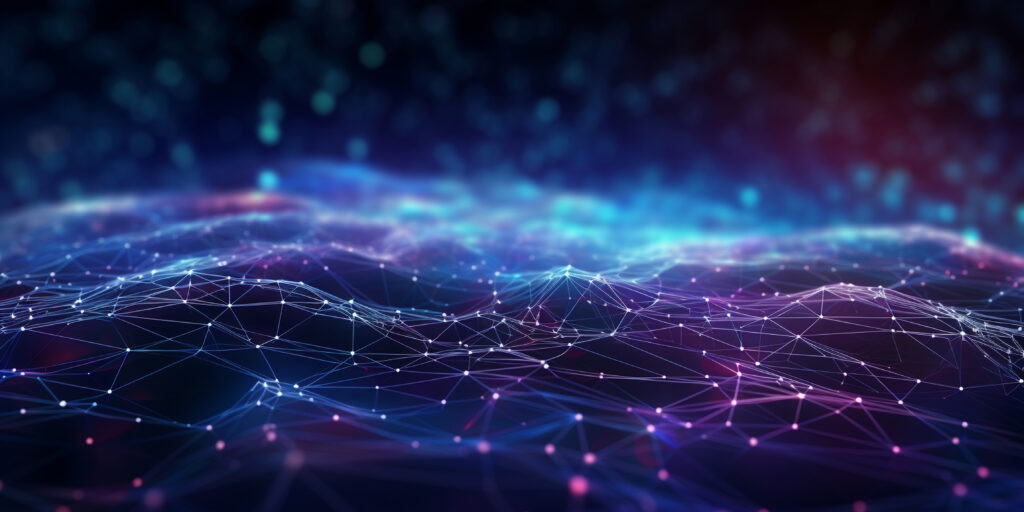
Moreover, the output of a quantum computer is probabilistic, which creates additional challenges. Classical computers give you a deterministic result – run the same simulation twice and you’ll get the same answer. But every time you run a quantum algorithm the output will be different. The result must be extracted from the distribution of the outputs (how many times you see 0s and 1s). To reconstruct the distribution accurately you must repeat the calculation many, many times which increases the overhead. This is another reason why some algorithms seemed very powerful a few years ago, but eventually were shown not to yield a systematic advantage over the classical ones we can already run on normal computers.
Does that mean that AI and quantum computing will be distant cousins, with little overlap?
Not at all. In fact, my colleagues and I have recently launched a petition2 to ask for funding at the European Union level for machine learning and quantum sciences. Machine learning is quickly becoming an essential tool to learn how to design and operate quantum computers nowadays. For example, every device is slightly different. Reinforcement learning techniques can analyse your machine and its particular patterns to help fit algorithms specifically to that device. There’s one company called Q‑CTRL3, for instance, that has been doing pioneering work in that field. Google’s quantum AI4 and Amazon’s Braket5 are two other leaders leveraging these ideas.
AI could also be very complimentary to quantum computing. Let’s take Microsoft’s Azure Quantum Elements, which used a combination of Microsoft Azure HPC (high-performance computing) and AI property-prediction filters to whittle down a selection of 32 million candidates for a more efficient rechargeable battery material to just 18 candidates. These were run through powerful, established, processing-intensive algorithms, which are quite limited because they consume a lot of energy, and can’t work with very complicated molecules. That is exactly where quantum computing could step in, in the near future.
I believe AI and quantum computing will be different components in a stack of tools — complementary but not compatible. We want to keep pushing those directions and many more by creating a joint team called “PhiQus” between Ecole Polytechnique (IP Paris) and Inria together with Marc-Olivier Renou and Titouan Carette.