Generative AI: what are the next steps?
- AI is moving forward a breakneck speed, and its pace of development is unlikely to slow.
- Some developments, like multimodal AI, AI agents, and AI-optimised chips are just around the corner.
- However, the development of AI is not yet profitable and is dominated by a few large commercial organisations.
- Bigger leaps like AI-powered robots and AI mentors are further away, but likely to happen.
- With these developments, regulatory bodies need to keep up.
AI has been a long time coming. But over the past couple of years, while it’s been in the public eye, it has seemed to advance at warp speed. Andrew Rogoyski shares his insights into what to expect next. What powerful new features can we expect are just over the hill for AI?
We should explain that when we use the term “AI”, we’re currently mostly focusing this discussion on “Generative AI” or “GenAI” which platforms like OpenAI’s Chat-GPT have brought to the world in the last two years. Further big advancements, being pushed forward by actors all around the world, are likely to come out soon. These already have a roadmap.
One of these is AI becoming increasingly multimodal. That means that large language models (LLMs) will learn and understand text, video, and sound and how they relate to each other. Some models are already breaching that barrier and reaching the markets. Single mode AIs like Copilot can generate images from text and vice versa. Sora can generate video from text. Runway and Pika Labs are also offering image-to-video generation. The newer Large Multimodal Models (LMMs) from OpenAI, Meta, Google and others can generate video from an image, text, and other data modes. For example, some GenAI models will answer text questions about the content of videos. Many industries are being affected with studios in Hollywood rapidly assessing what this could mean for the movie industry. One of the downsides of this powerful technology is that you can create fairly intricate deepfakes with smaller budgets.
Another big, expected advance will be AI becoming an invisible tool. Instead of having to log on to a dedicated platform on a computer or phone, we’ll be able to converse with our cars, phones, and appliances, and get very natural answers. There are several companies working on this, Apple with Apple intelligence, Google with Google AI, Amazon with Alexa, and others.
The next step then is having AI act as a sort of agent on your behalf, allowing it to book in trips, hotel stays and so on. At this point, GenAI isn’t very good at planning. That’s what OpenAI and others are working on, getting GenAI that can break down a problem into steps and take action on those steps. The question is, then how much authority do you give an agent to act on your behalf? It seems likely that such agents will be interacting with other agents, leading to entire AI discussions and negotiations taking place without human intervention.
Another fairly big development will be the improvement of AI retrieval. That may sound boring, but it’s really exciting in terms of productivity. Corporations collect thousands of documents containing customer interactions, bids, policies, procedures, and other useful information. However, retrieval of such information is generally poor. GenAI may be the solution to the corporate “knowledge management” problem. Wouldn’t it be wonderful to be able to ask your laptop: “What was that big bid we did three years ago where we partnered with that bank?” and have it infer the right answers and give you a summary rather than a string of documents you have to read through?
Of course, before we can do this, we need to tackle AI hallucination, which is the false information generated by AI.. We have developed a technology that will hallucinate images, sounds, poetry, and so on. But we are less keen on it hallucinating the company accounts or a medical record. The trick now will be to take that really nice conversational interface and link it to hard facts. Generative AI can create nonsense, which can be a big problem. Recently, Air Canada faced a small claims court case1 from a passenger who tried to retroactively apply for a refund on his ticket after checking the company’s bereavement policy on their AI-powered chatbot. The AI hallucinated that passengers could claim back money within 90 days of travel, which isn’t in the company’s policy. The court sided with the passenger.
Part of the move forward with AI will be limiting its cost, right?
Yes, cost of running these models today, in terms of energy, cooling, and computing power, makes them unsustainable, both commercially and in the context of the climate crisis. Companies are likely to move from the existing graphics processing units (GPUs) to hardware designed around AI applications.
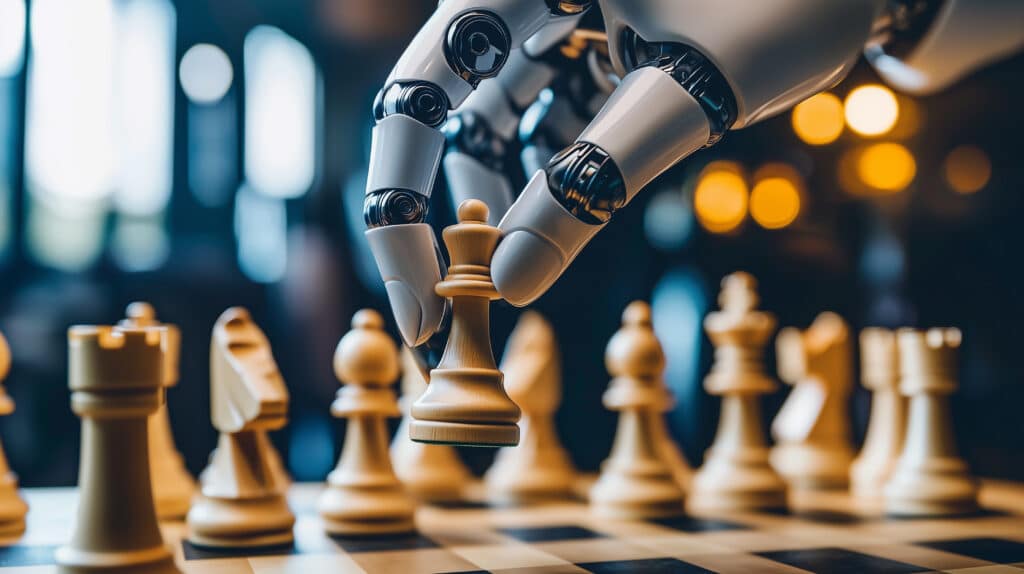
Apple have a “neural processing unit”, Google have a “Tensor processing unit”, Microsoft, IBM, Amazon, Samsung and others are all developing specialised hardware that can deliver performance improvements hundreds or thousands of times more efficient than GPUs and CPUs. These chips are massively parallel and optimised for the matrix operations at the heart of machine learning algorithms.
New chip architectures are also being proposed to run these models with very low energy. That’s the case for IBM’s North Pole AI chip2, for instance, which promises to reduce the power for typical applications by a factor of 253. Google is also working on its Tensor Processing Unit to accelerate AI processing and Groq’s Language Processing Unit is also showing promise.
Then there are more esoteric architectures, such as the neuromorphic chips. These are designed to support so-called spiking neural networks — computing models that mimic the way human brains work. Those are mostly in the academic domain at the moment, but they are starting to move into other areas.
What about the fact that AI is that it is so heavily dominated by a few commercial entities at the moment?
Currently, there is a big debate about opening up LLMs to open source. Due to the scale of operations needed to develop LLMs and LMMs, commercial organisations have been very much at the forefront of development. Around 80–90% of them are developed by commercial organisations. That means that the technology has remained mostly in the hands of its proprietors—with some notable exceptions like Meta’s LLaMA and Mistral’s Large and Codestral, which were made open source early on. There are also open-source community LLM/LMMs like Platypus, Bloom, and Falcon.
On the one hand, more people experimenting and playing with the technology could trigger new advances, expose vulnerabilities, and so on. On the other hand, there are people who will misuse that technology. There are currently fail-safes built into most of the models so that people can’t do whatever they want, however they’re relatively easy to circumvent. And some open-source models are available in their “raw” state with no guardrails. We can expect that open source GenAI will continue to grow. This goes hand in hand with the push to develop smaller, more sustainable, models that don’t require hundreds of millions of dollars to run.
What issues can there be expected in terms of misuse of such new technology?
Cybersecurity will continue to be a huge issue. Criminal organisations are quickly learning to harness this technology for nefarious purposes. They have already started using generative AI to streamline online surveillance, to mine historical data for vulnerabilities, or to automate attacks with fake texts. Scammers are also using deep fakes to swindle money out of companies. The Hong Kong police recently made six arrests4 in relation to an elaborate scam that robbed UK engineering firm Arup5 of $25 million. One of the company’s workers was pulled into a video conference call with what he thought was his chief financial officer. This turned out to be a deepfake video. Deep fakes are also targeting voters’ intentions with misinformation. It’s a very dangerous trend and real threat this year, 2024 running the most elections that humans have ever held in our history.
While cyber scammers will continue to improve, defenders on the other side are also learning, using generative AI and other forms of AI to find attackers. There’s this constant cycle of attack and defence in the cybersecurity world.
There is also a big discussion around the use of AI in a military context. AI is already used to analyse satellite imagery or provide navigation for drones, but it is not yet known to be used to take human life. At this point, it’s still cheaper not to put AI on drones even if it is technically feasible. And that’s a very important line, in my view, not to cross. We don’t want to enter a world where you have been fighting a machine speed and your adversary is an AI – it’s then a short step to the dystopian worlds of James Cameron’s Terminator movies or the Wachowski brothers’s Matrix series.
We are seeing some movement from regulatory bodies, where do you expect that to go?
There is regulation starting to emerge. The European Union AI Act came into force6 in August 2023, with the details being finalised in April this year. Everyone will be watching what impact the EU legislation has. A US presidential order was published7 in October introduced a long list of controls, including statutory reporting above a certain level of computing and networking power. We can expect more legislation to come out of the US, UK and other countries soon.
Science fiction has a disturbing habit of becoming science fact.
Still, unless you hold those developing AI accountable, that regulation will only go so far. At the moment, it’s free reign. If the technology puts millions of people out of jobs or causes a mental health epidemic, corporations can shrug their shoulders and say they don’t control how people use the technology. On the other hand, if large corporates are the only organisations willing or able to invest the tens of billions necessary to develop these AI systems, nobody wants to stall this and risk falling behind other countries.
We need legislation and regulation where organisations and individuals are accountable for the impact of their technologies. That would make them think carefully about how their technology is going to be used and put the onus on them to properly explore and test the impact of their technology. You can see this is an area of tension for some of the GenAI companies, for example, OpenAI has lost several leading people8 from their company, each of whom is hinting at the lack of oversight in GenAI development.
Anything else we should be looking out for?
There are advances that are over the horizon, but you can see that they will come. And those will be very significant. I think the convergence of quantum computing and AI will be interesting. Some companies like IBM are now bringing forward their roadmaps on quantum computing. IBM is now foreshadowing 200 qubits and 100 million computing gates by 20299. That is very powerful technology that may allow AI to learn in real-time and that gets really exciting.
Over the past 12 months or so, people have been applying the large language model approach to robotics, so-called Vision Language Action models, or VLA. In the same way that we’ve built foundation models for text and images, we may be able to build them for robotic perception, action, and movement. These aim to get to a place where, for instance, you can tell a robot to pick up a banana and it’s got enough general knowledge to not only spot the banana with its sensor but figure out what to do with it, without requiring specific algorithmic input. It’s quite an interesting advancement in robotics because it also allows the AI to learn from physical and real-world experience.
AI mentors could be another big thing. AIs are already being used to generate learning material, but you can imagine a world where an AI scans your CV, and is able to suggest training, reading material, and so on. AIs could also act as tutors, guiding you through education, suggesting ways of learning, doing exams and assessments, and following your development. Schools are already piloting the use of GenAI as tutors, for example, David Game College in London10 is trialling an accelerated GCSE in which students are only taught by AI. You’re getting into and changing the entire educational loop then.
The question might then be: why you would go to university? Why would you even go to school, apart from its social benefits? It could fundamentally change how we learn and teach. Some may be concerned that we start to build new education systems that are dependent on US tech companies, rather than in-country qualified human beings.
What kind of timescale are we thinking of for these advancements?
I think if we’ve learned anything from the last couple of years, it’s that things can happen really fast. Things are never as far-fetched as we would imagine it to be – Science fiction has a disturbing habit of becoming science fact. I would say much of it is disturbingly close.
Now we need to start thinking the consequences of this. What is humanity’s role in this future? What do economies look like if humans are taken out of the equation? What does truth and democracy look like when anything can be faked? What does education, the foundation of our modern quality of life, look like in the future? These are very big, fundamental questions that I think no one has the answer to at the moment.