Biomimetics is no stranger to the rapid progress and breathtaking performance of today’s algorithms. But the IT community is still struggling to integrate the true power of the living world: its energy efficiency.
Biomimetics has been part of the history of algorithmics since its earliest developments. “In 1964, the first neural network, the perceptron, was already biomimetic. It sought to reproduce the electrophysiological properties of neurons, their excitability and ability to transmit information”, explains Clément Viricel, lecturer at Lyon University. Each neuron receives data, evaluates it and produces a result according to the function specified in the algorithm. This process constitutes the “activation” of the artificial neuron, just as a neuron is activated in the brain by nerve impulses. In the perceptron, the neurons were connected in a single layer. It was by multiplying the layers of neurons that it processed the flow of information.
Neural networks
From the 1990s onwards, training algorithms adopted these neural networks in an attempt to reproduce the way in which humans learn. “Neural networks are biomimetic because they learn by error, rather like humans or babies. Plasticity can be represented by matrices whose elements are weighted according to success. The coefficients play the role of reinforcement between neurons”, explains Laurent Pujo-Menjouet. Clement Viricel adds, “For example, when learning a language, humans often discover the meaning of a word through context. Semantics play a crucial role. This is what neural networks began to do, by being trained with texts in which a word was missing. Then they were optimised by backpropagation”. In other words, by correcting the weights of the input neurons according to the output results. “But this process is a black box, where the variations in weighting that enable the algorithm to evolve are not visible,” adds Clément Viricel. And we know that it’s difficult to trust a process if you don’t understand how it works. These methods are a major headache for underwriters in charge of products that incorporate them, such as autonomous vehicles1 or diagnostic assistance systems2.
Biomimicry contributes to the development of a large number of algorithms. These include so-called “genetic” algorithms, which are based on phylogenetic trees for calculation purposes, and enable the most relevant result to be selected according to several methods (by rank, tournament, adaptation, etc.). Systems such as these have been deployed in search of optimums, but also in the development of games, such as the famous Super Mario, to rank the players amongst themselves. There are also convolutional neural networks, inspired by the human visual network. “Its developers wanted to reproduce the way in which the eye analyses an image. It’s a square of neurons, which scan the image to capture the pixels before reconstructing it in its entirety”, explains Clément Viricel. This tool is renowned for having surpassed the expert eye, particularly in the diagnosis of melanoma3. How does it work? “During the training period it extracts characteristics such as ‘tumour shape’ and ‘tumour size’. Then it looks for these characteristics to recognise a particular object”, explains Clément Viricel.
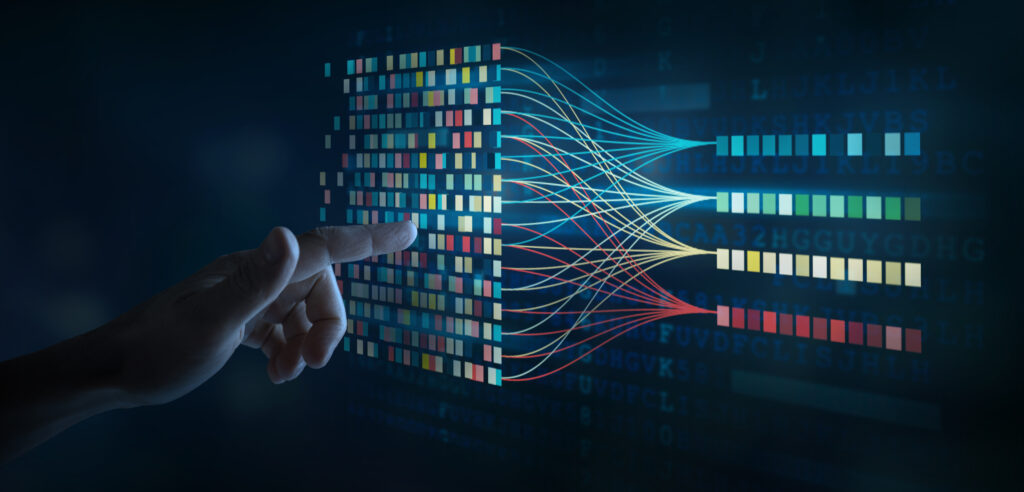
These biomimetic algorithms are applied to all subjects, as exemplified by recurrent neural networks. “They are used to analyse data sequentially or over time. It is widely used for automatic processing of texts, by taking word order into account. Dense layers are recurrent so that the network doesn’t forget what it has done before”, explains Clément Viricel. Such networks have been used to build machine translation tools. A first recurrent network “reads” and encodes the text in the original language, a second recurrent network decodes the text in another language, all of which takes time and energy. “They require a lot of energy to train”, admits Clément Viricel.
Transformers
So, we need to be able to learn faster. Specialists therefore devised a way of reproducing lexical dependency: when a human learns a text, they implicitly know what each pronoun refers to. This makes sentences lighter. “To reproduce this, we had to add an extra layer of neurons, the attention layer. And this is the parameter on which the latest biomimetic evolution has taken place”, explains the specialist. The inventors of these new artificial intelligences have titled their article “Attention is all you need”. In fact, their network consists of just 12 layers of attention and an encoder/decoder system. These networks are called “transformers”, and are the models used by Google’s Bert and Bloom, the Hugging Face start-up founded by three Frenchmen. (Chat-)GPT is a direct descendant of the transformers, although it has only the decoder and no encoder.
This whole story is a good example of how biomimetics has fed into algorithmic innovation, while forgetting one of the essential characteristics of living organisms: energy efficiency. For example, training GPT‑3 Cat required 1.287 MWh and emitted 552 tonnes of CO24 “Until now, developers haven’t been at all interested in the energy footprint of their networks”, admits Clément Viricel. “It’s a skills problem. The people who design the algorithms are not the same people who build the physical components. We forget the machine aspect. Recent tools consume an enormous amount of power… and the next systems, TPU or HPU, won’t be any more environmentally friendly,” explains the specialist.
The change could come from the next generation of programmers. “We are seeing the emergence of a movement in the community to address this issue. On the one hand, this is due to the need to optimise energy consumption, but also for ethical reasons. For the moment, the improvements are purely mechanical, based on energy transfer”, explains Clément Viricel. But other avenues are emerging, such as zero-shot shock zero-shot learning algorithms: “They work without training, which saves on the cost of learning”, adds the specialist. It remains to be seen whether their performance can compete with that of their predecessors to produce totally biomimetic systems.