Understanding short-term memory through neuronal plasticity
- Synapses, not neurons, play the main role in working memory.
- To simplify the analysis of neural networks, early studies considered neurons to be ‘fixed’, thereby obscuring synaptic plasticity.
- Researchers at Columbia University updated the theory by including synaptic and neuronal dynamics.
- They discovered that synaptic dynamics can modulate the overall behaviour of neural networks, speeding up or slowing down neuronal activity.
- A new behaviour, called ‘frozen chaos’, was identified, where synapses create fixed patterns of neuronal activity, potentially crucial for working memory.
- There is still room for improvement in this model: neuroscientists now want to incorporate certain biological properties of the brain to make it more realistic.
What role do neurons and synapses play in working memory? This is a question that neuroscientists have long pondered. Until now, it was thought that neuronal activity dominated, with synapses only involved in the slower processes of learning and memory. But researchers at Columbia University have now developed a new theoretical framework that predicts that synapses rather than neurons are more important. Their new model might lead us to an alternative mechanism for working memory in the brain, they say.
The human brain is made up of around 100 billion neurons. Each neuron receives electrical signals from other neurons via thousands of tiny connections called synapses. When the sum of the signals emitted by the synapses exceeds a certain threshold, a neuron “fires” by sending a series of voltage spikes to a large number of other neurons. Neurons are therefore “excitable”: below a certain input threshold, the output of the system is very small and linear, but above the threshold it becomes large and non-linear.
The strength of interactions between neurons can also change over time. This process, known as synaptic plasticity, is thought to play a crucial role in learning.
With and without plasticity
To simplify things, early studies in this field considered that neuronal networks were non- plastic. They assumed that synaptic connectivity was fixed, and researchers analysed how this connectivity shaped the collective activity of neurons. Although not realistic, this approach has enabled us to understand the basic principles of these networks and how they function.
David Clark, a doctoral student in neurobiology and behaviour at Columbia University, and Larry Abbott, his thesis supervisor, have now extended this model to plastic synapses. This makes the system more complex – and more realistic – because neuronal activity can now dynamically shape the connectivity between synapses.
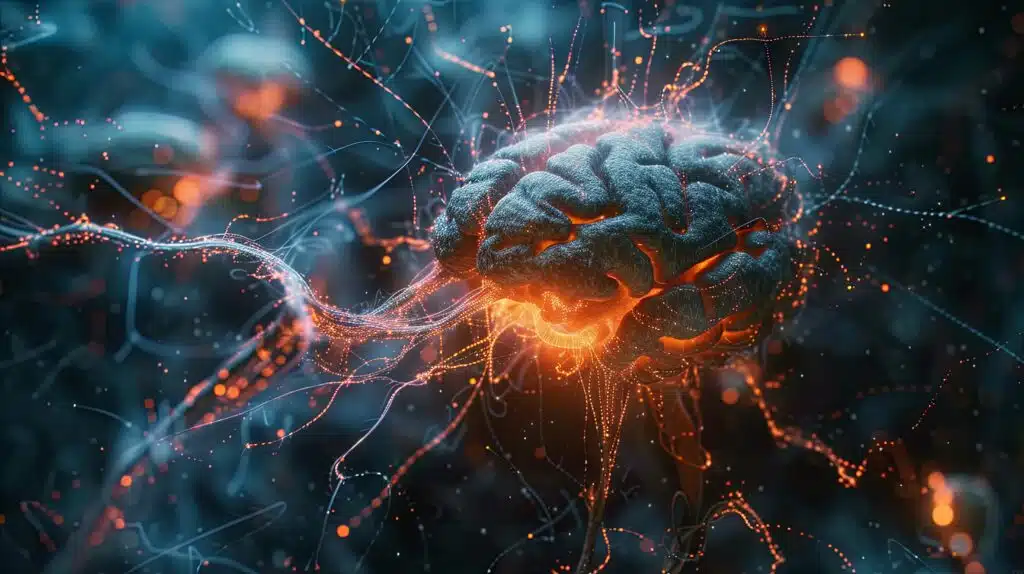
The researchers used a mathematical tool known as dynamic mean field theory to reduce the “high-dimensional” network equations of the original model to a “low-dimensional” statistical description. In short, they modified the theory to include synaptic and neuronal dynamics. This allowed them to develop a simpler model that incorporates many of the important factors involved in plastic neural networks. “The main challenge was to capture all the dynamics of neurons and synapses while maintaining an analytically solvable model,” explains David Clark.
Synaptic dynamics become important
The researchers found that when synaptic dynamics and neuronal dynamics occur on a similar time scale, synaptic dynamics become important in shaping the overall behaviour of a neural network. Their analyses also showed that synaptic dynamics can speed up or slow down neuronal dynamics and therefore reinforce or suppress the chaotic activity of neurons.
Above all, they discovered a new type of behaviour that appears when synapses generate fixed patterns of neuronal activity in networks. These patterns appear when plasticity is momentarily deactivated, which has the effect of “freezing” the states of the neurons. This “frozen chaos”, as the researchers call it, can help to store information in the brain and is like the way working memory works.
The scientific challenge of our study was to translate this intuition into equations and results.
“This research topic came about when Larry Abbot raised the idea, while chatting in his office, that dynamic synapses play just as important a role in neuronal computation as neurons themselves,” explains David Clark. “I found this idea very interesting, because it flips the typical view of neurons as the dynamic units, with synapses only being involved in the slower learning and memory processes. The scientific challenge in our study was to translate this intuition into equations and results.”
“The new model provides a possible new mechanism for working memory,” he adds. “More generally, we now have a solvable model of coupled neuronal and synaptic dynamics that could be extended, for example, to modelling how short-term memory is consolidated into long-term memory.”
David Clark and Larry Abbott now hope to make their model more realistic by incorporating certain biological properties of the brain, including that neurons communicate via discrete voltage spikes. Other important features, such as the fact that neurons are patterned in specifically structured connections, will also have to be taken into account, they add.
Isabelle Dumé
Reference: D. G. Clark and L. F. Abbott, “Theory of coupled neuronal-synaptic dynamics,” Phys. Rev. X 14, 021001 (2024).