New algorithms predict eyesight loss caused by ageing
- Opacification of the eye is a phenomenon that affects about 10 million people worldwide.
- Today, clinical evaluation tools do not allow for early diagnosis or quantitative monitoring of corneal pathologies: the doctor must interpret the result.
- Maëlle Vilbert is developing an efficient image analysis method to avoid potential interpretation bias.
- Artificial Intelligence (AI) models, if properly trained, can detect problems that humans would not see with the naked eye.
- Using AI would allow doctors to spot potentially pathological situations very quickly and ensure better patient care.
“The World Health Organisation [WHO] estimates that 80% of blindness is preventable,” explains Maëlle Vilbert, a doctoral student at École Polytechnique (IP Paris). More than 10 million people in the world are affected by a visual handicap due to the loss of transparency in the cornea. Although, this issue remains less common than cataracts (opacification of the crystalline lens), and glaucoma (linked to excessive intraocular pressure), loss of corneal transparency remains one of the main sources of deterioration, or even total loss, of vision.
The eye is one of the most complex organs in the human body. For it to function properly, and therefore for clear vision, it must be composed of several healthy components. However, as we age, these elements deteriorate in many people. Thus, even if the causes of blindness are diverse and varied, one of the main causes is the opacification of the cornea. This naturally transparent lens, which covers the iris at the front of the eye, allows light to pass through. Its clouding directly affects a person’s vision – a phenomenon that affects about 10 million people worldwide.
Opacification of the eye is a phenomenon that affects about 10 million people worldwide.
Today, corneal transplantation is the most common type of transplant in the world. And whilst, it remains unavoidable to treat advanced stages of corneal opacification, it is better to prevent it entirely. Indeed, aside from the risks associated with the operation, there is a serious shortage of corneal grafts worldwide, with an average of 1 donated cornea for every 70 needed.
According to Maëlle Vilbert, the clinical tools for assessing corneal transparency remain qualitative and/or operator-dependent, which does not allow for early diagnosis or quantitative monitoring of corneal pathologies. “Practitioners analyse optical coherence tomography (OCT) images with the naked eye,” explains the researcher. “There is no standardised method for extracting properties directly related to the tissue. This leaves room for the doctor’s subjectivity. If the problem is not obvious, he or she may not be able to see it.”
“Even so, this imaging method records the image of each cornea that has been examined,” explains Maëlle Vilbert, “which provides us with an enormous database”. Vilbert is working on this data for her thesis to develop a method of image analysis that will enable a physical measurement of corneal transparency, so as to avoid potential biases in the interpretation of images.
“By understanding its transparency, we understand its opacification”
Transparent tissue is unusual in nature, but this characteristic of the cornea can be explained. The stroma is a connective tissue that makes up 90% of the thickness of the cornea. It is composed of nanoscale collagen fibrils whose diameter and spacing within stratified lamellae reflect a localised orderly organisation. This local order gives rise to destructive interference in the tissue in all spatial directions except direct transmission, hence the remarkable transparency of the cornea. Only the light signal transmitted directly through the cornea and lens allows images to be formed on the retina.
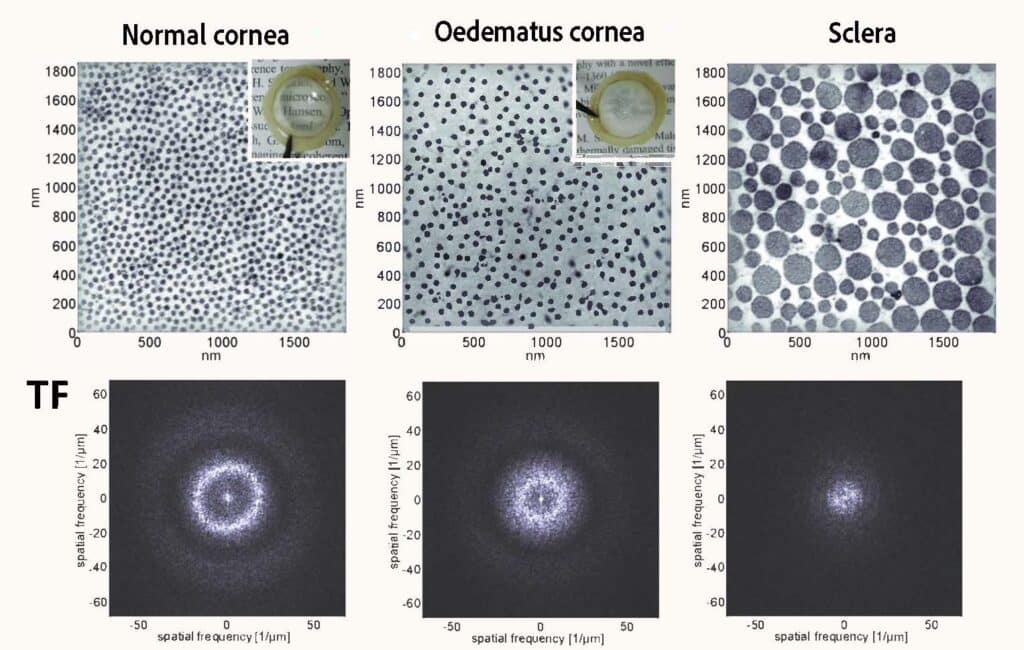
“A light wave can be transmitted, absorbed, or scattered by a medium,” says Maëlle Vilbert, “and the cornea does not absorb anything, so it either transmits or scatters the light. As soon as the scattering phenomena become too great, the cornea becomes opaque, and its transparency is lost.” Hence, it is this orderly organisation of the collagen fibrils making up the stroma that makes corneal transparency possible. If its composition becomes disordered, as is the case in the sclera – the white of the eye – where the diameter of the collagen fibrils is not constant, light is no longer transmitted directly into the eye.
“Corneal oedema is one of the causes of opacification,” she adds, “because it causes the appearance of micrometric aqueous interstices between the collagen lamellae of the stroma, often called ‘lakes’, which scatter the light.”
Coupling physics and AI for a more reliable and accurate analysis
Maëlle Vilbert’s project is based on the hypothesis of a homogeneous corneal stroma in order to characterise its transparency using physical parameters. “A heterogeneous environment would cause local variations in the attenuation of the OCT signal,” Vilbert explains. “By statistically validating the consistency of the corneal stroma, we can quantify its transparency using a single percentage of transmitted coherent light. The aim is to standardise methods for analysing OCT images. This allows us to distinguish between a normal cornea and a pathological cornea with low scattering, which is difficult to detect with current clinical tools.”
However, corneas with localised opacities cannot provide a single transparency parameter. “We have also adopted an approach of automatic classification of clinical images to detect certain corneal inflammations, such as in Fuchs’ dystrophy or corneal haze after refractive surgery. AI models, if properly trained, can detect problems that humans would not see with the naked eye.”

“Of the various parameters used by the team to train the AI model, one parameter (“sigma”) alone has a classification accuracy of 93%: it reflects the depth of the fire zone. The other 8 parameters increase the classification accuracy to 97%. These parameters can still be interpreted by practitioners,” she insists, “which is essential for the positive reception of this type of digital diagnostic tool.” Doctors could thus use this AI to detect certain symptoms at an early stage, especially when they are not visible to the naked eye and ensure follow-up over time for better patient care.
The methods developed by this team are complementary tools to traditional slit lamp and OCT diagnosis. Being able to assess the transparency of the cornea with such accuracy in the given percentage allows potentially pathological conditions to be identified very quickly. It also makes it possible to establish an effective follow-up over time, as it is quantitative. Thus, it would be possible to intervene early and avoid the need for invasive treatments such as a corneal transplant.
AI models, if properly trained, can detect problems that humans would not see with the naked eye.
“There is a real challenge in this analysis technique,” explains Maëlle Vilbert, “given the ageing population and the fact that 80% of cases of blindness are preventable. More accurate diagnosis, both at the time and in the long term, means more effective prevention and better patient care.” The tool designed by the researcher and her team is easy to use due to its automation. Thus, after a short training course, the diagnosis of corneal transparency is affordable for people who are not experts in the field.
These methods coupling AI with the physics of light propagation in human tissues have a promising potential for the design of clinical diagnostic tools. They could, for example, be transferred to the diagnosis and monitoring of cataracts, a condition that accounts for more than half of all visual impairments worldwide.
Pablo Andres
Further reading
For more details on the research, here is the work of Maëlle Vilbert: https://www.theses.fr/s242473