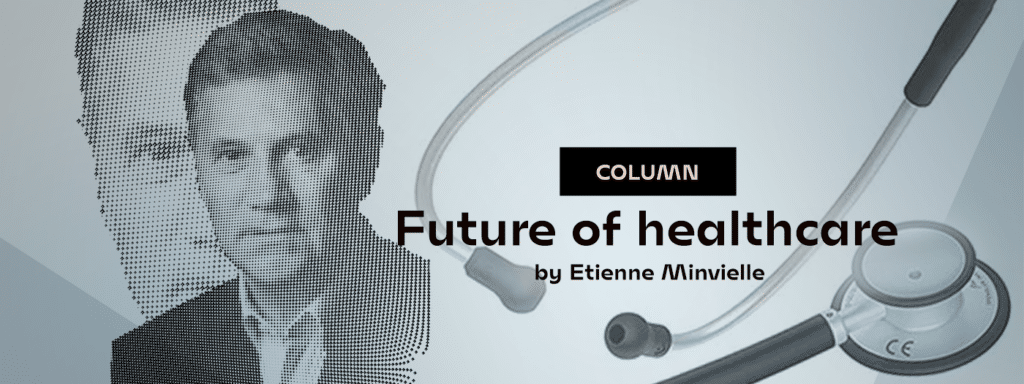
Epidemic risk prediction models help to analyse the temporal and geographical evolution of epidemics. They existed well before digital algorithms. However, since the advent of Big Data, these models have evolved considerably, raising several questions. How reliable is the prediction? How can we assess our ability to collect data? What is the role of these models when it comes to taking action?
Predictive models: before and after Big Data
Since the 20th Century, many models have been developed and have proved their worth. The SIR mathematical model, created in 1927, forms the basis of most epidemiological models. It is based on flows between the compartments of the susceptible (S), the infected/contagious (I) and those removed from the transmission chain (R) – i.e. people who have been immunised or have died1.
An infection is said to be an epidemic when the number of sufferers increases over time, i.e. when the number of new R0 infections is positive – as we saw during the COVID-19 crisis. In short, this means that each case on its own generates at least one case.
At the same time, other models – ARIMA2 and SARIMA3, for example – are not based on the SIR model, but on a “time series”. They assume that what happened in a previous series will happen in future episodes. These models are effective for seasonal events such as influenza.
With the emergence of Big Data, new predictive models have appeared. These can be used to anticipate epidemics so that humanitarian aid can be concentrated in the area at risk, at the key moment4. In recent years, several practical applications have proven their worth. For example, to combat Ebola in Africa, Médecins Sans Frontières has built health centres in areas of high traffic flows, identified using data from telephone operators5. Capturing a new kind of data in large numbers opens new treatment possibilities. In this respect, algorithmic prevention is effective.
Unpredictability and action: the challenges of these Big Data models
At present, it seems that models predict more for the short or immediate future than for the longer term. None of the recent diseases (COVID-19, Zika, West Nile, Chikungunya) has ever been predicted – we always caught up once it was already there. When we try to predict the risk of an epidemic occurring, models tend to overestimate risk.
In January 2013, for example, the Google Flu Trends interface predicted – wrongly – a serious flu epidemic in New York. Based on this prediction, large-scale preventive measures were launched, which then proved to be completely useless. Similarly, the CDC in Atlanta (the US Centre for Disease Control and Prevention) predicted that there would be over a million cases of Ebola in Liberia, but fortunately there were only a few tens of thousands of cases.
On the other hand, models are effective for tracking the development of epidemics over the short term. Google Flu Trends has demonstrated this on many occasions. Another example: during the COVID-19 pandemic, Google Verily, the University of Geneva and the École polytechnique de Lausanne-Zurich were able to predict short-term epidemic waves.
The other problem with models is the relationship between results and action. On the one hand, a model produced on a national scale does not necessarily have sufficient power to assess a local situation. During the COVID-19 pandemic, for example, local models were developed in Martinique in addition to the national Pasteur model. This very simple model forecast the number of COVID beds needed over 14 days if there was no containment. During the 4th wave (the largest), the model predicted that 700 COVID beds would be needed, proving to be fairly reliable since 600 beds were actually used. This model was effective in anticipating the impact on day hospital services for chronic diseases and in opening beds accordingly, demonstrating the need to supplement global analyses with others that are located and adapted to the local context.
On the other hand, regardless of the model chosen, its reliability and its adaptation to a local context, prediction alone cannot govern action. The COVID-19 pandemic showed that many people were reluctant to be vaccinated, with varying profiles and motivations. In China, elderly people were discouraged by doctors who cited their fragile health. In the case of the African-American and West Indian populations, it was rather a lack of confidence in the Western powers that appeared to be the reason for resistance to vaccination. There were therefore many reasons for the reluctance to vaccinate, and these were independent of the question of prediction.
Generally speaking, these challenges reveal that the transition from prediction to preventive action is not linear and sequential. Other socio-economic factors come into play, underlining the importance of placing predictive models in the context of their use.
The future of prediction: towards multidimensional, unified integration?
The contribution of Big Data seems likely to improve matters. Multi-level predictive models could be developed by combining more epidemiological expertise, Big Data and algorithmic processing. Such modelling, in which each layer could contribute to accuracy, concerns a variety of data: satellite imagery, biological data, economic and social data, health monitoring, etc.
This presupposes more dynamic data collection and sharing. In this respect, the uptake of data in France during the last Covid crisis showed that the approach is not yet spontaneous. It would have been – and still is – desirable to set up a unified data warehouse, so that experts can draw on it for the data they need. To achieve this, we need to learn how to organise the sharing of existing data. This is a major challenge if we are to make progress in the algorithmic prevention of epidemic risks.