“A few years ago, we came together as part of a group of researchers from the Management Research Centre at Ecole Polytechnique working in the healthcare sector,” explains Étienne Minvielle, CNRS research director (IP Paris). “One of the objectives was to think about how to bring technological innovations into dialogue with the needs of the healthcare system.” This meeting launched a series of seminars on algorithmic prevention. “Two years ago, I personally didn’t know much about this topic,” he admits. “To tell the truth, I didn’t really see what could be said about it. However, after having led these seminars, I realise how important this subject is for improving prevention.”
Because, although initially little was known about this subject, even among professionals in the field, these discussions have highlighted the fact that algorithmic prevention affects almost all areas of medicine (oncology, geriatrics, psychiatry, neurology, etc.).
From theory to practice, the algorithm prevents disease
From digital twins to the prevention of epidemics, ageing well and augmented psychiatry, these seminars show that algorithmic prevention is not limited to a specific field. It paves the way for a systemic transformation of medicine, linking technological innovations to societal issues. Health prevention can now take two forms: conventional prevention, which is aimed at a large group of the population, and so-called algorithmic prevention, which is more personalised. “Algorithmic prevention differs from conventional prevention in that it is personalised and accompanied by dynamic monitoring,” says Étienne Minvielle. “This involves the collection of substantial data on genetic, but also socio-economic and behavioural, factors.”
___________________________________________________________________________________________
Read also: How digital technology will personalise healthcare
___________________________________________________________________________________________
Once this data has been collected, coupled with a more in-depth knowledge of the development of the disease, it becomes possible to make better predictions. “For example, the Interception programme at Gustave Roussy is based on the observation that 40% of severe forms of cancer could have been identified earlier by these algorithmic processes,” he explains. “Tests are thus carried out to identify genetic polymorphisms, i.e. combinations of genetic mutations, in people identified as being at risk. Coupled with an analysis of environmental risk factors, they make it possible to predict the risk of cancer occurring and to “intercept” it even before it can develop, thanks to personalised monitoring.”
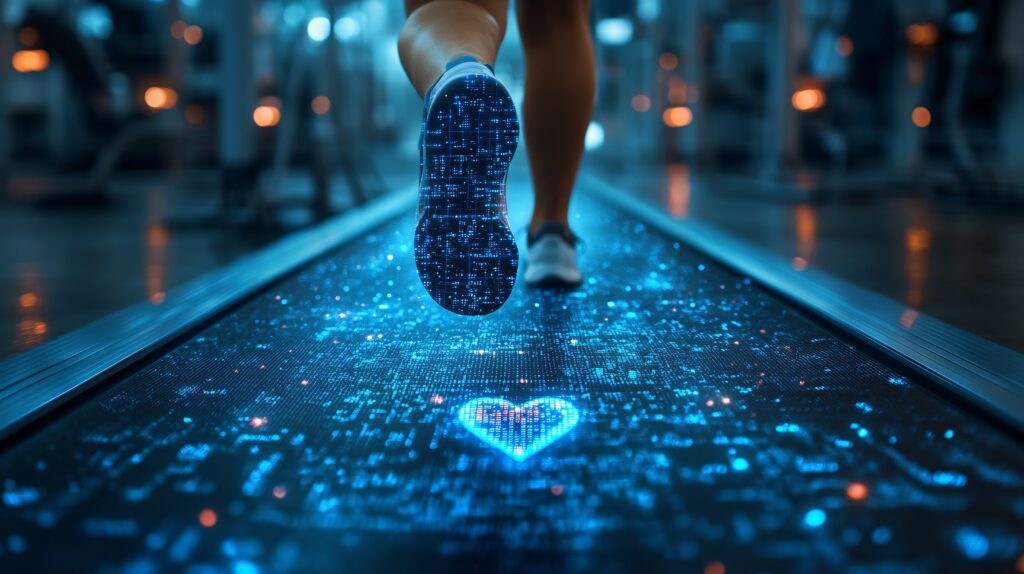
40% of cancers could therefore be avoided, as Fabrice Barlesi, CEO of Gustave Roussy, is well aware: “Once we know this, we cannot fail to recognise the importance of prevention. But we must also ask ourselves why prevention is not working today – smoking is a case in point. Moreover, our screening programmes, i.e. early detection of the disease, are also struggling. To remedy this, we will need to be able to identify the people at highest risk with a view to intercepting this disease.”
A similar pattern is found in the prevention established by the ICOPE programme in its quest for healthy ageing, in the prevention of cognitive decline (such as with Alzheimer’s disease), and in other conditions (cardiology, mental health, well-being).
The science behind the algorithm
However, examples of the application of this type of prevention highlight its dependence on our scientific, technological and organisational advances. “Today, we can see that innovation is a major lever for contributing to an effective prevention policy,” says Lise Alter, former director general of the Health Innovation Agency. “And, between an ageing population, which means an increase in the prevalence of chronic diseases, the diverse and varied challenges of climate change, and the limiting factor of human resources in the healthcare sector, we are going to have to face major challenges that will require the transformation of our healthcare system.”
And it is these major challenges that make the promises of algorithmic prevention so appealing. “When we talk about “transformations” it means “changes” in our financing models, which are mainly based on curative rather than preventive care. Evaluation and also demonstration of value – requiring application on a population scale sufficient to have a power of demonstration.” Above all, the demonstration of effectiveness must not stop at the clinical aspect but must also focus on the impact of such a change on the organisation of care or on the quality of life of healthcare personnel. “These are therefore much broader considerations than the simple clinical impact on the patient, even if this remains a fundamental point.”
For his part, Nicolas Revel, Director General of AP-HP, Assistance Publique – Hôpitaux de Paris, is clear: “I am convinced that we are going to have to turn prevention, which is a great idea, into a reality. This will require the removal of a few obstacles, both economic and financial. And, indeed, at a time when we are seeking to reduce expenditure, innovation will be the key to convincing decision-makers to invest and to successfully implementing it.” One of the lines of attack could also be to demonstrate effectiveness, for primary as well as secondary and tertiary prevention. “Although primary prevention requires long-term investment, it creates benefits that have an impact on secondary and tertiary prevention. This would enable us to bring the healthcare system closer to the patient.”
This transformation cannot happen overnight. As Lise Alter rightly points out: “Before any changes can be made, sufficient evidence is needed to provide these elements of objectivity.”