High-frequency trading: what are the risks and the returns for financial markets?
- High-frequency trading refers to buy and sell orders made on financial markets over an extremely short timeframe.
- Trading is automated using algorithms and computers, enabling it to react very quickly to market events.
- On the equity markets, around 2/3 of all transactions are carried out by high-frequency traders.
- If the investment is substantial, risk-taking is minimal, as reaction time is optimised to make the most of opportunities.
- This system can be overridden by algorithms, due to bugs, or to the detriment of algorithms, due to hacking.
Hi! PARIS is the new Center on Data Analytics and Artificial Intelligence for Science, Business and Society created by Institut Polytechnique de Paris (IP Paris) and HEC Paris and recently joined by Inria (Centre Inria de Saclay).
What is high-frequency trading?
The term refers to the placing of buy and sell orders on the financial markets over an extremely short time frame, on the order of nanoseconds, generally in reaction to events. For example, when the Fed decides to cut interest rates, high-frequency traders will place orders a nanosecond after the macroeconomic announcement is made.
Who carries out these transactions?
Obviously, humans can’t react on this time scale. High-frequency trading is characterised by two phenomena. Firstly, it is automated trading: it requires the use of algorithms and computers to place orders. Secondly, it must react extremely quickly to market events, whether macroeconomic announcements or changes within the market such as price movements.
This automation is nothing new.
If we look at the Paris Stock Exchange, for many years we had this image of traders shouting their orders around the trading floor. This has not been the case since 1986, when the trading floor was replaced by a computer RAM. Since then, traders have been carrying out their transactions all over the world via online terminals. This move towards automation began in the late 1960s and ended for equity markets in the early 1990s. This automation led to so-called algorithmic trading and, over the last twenty years, to high-frequency trading.
How much of this is high-frequency trading?
On the equity markets, it’s estimated that around two-thirds of transactions are carried out by high-frequency traders. This may seem high, but it’s not all that surprising. It’s a bit like supermarkets: while they account for a large proportion of consumer transactions in France, they are merely intermediaries. High-frequency traders are not the final holders of securities, but the principals. They generally only hold the securities for a very short time.
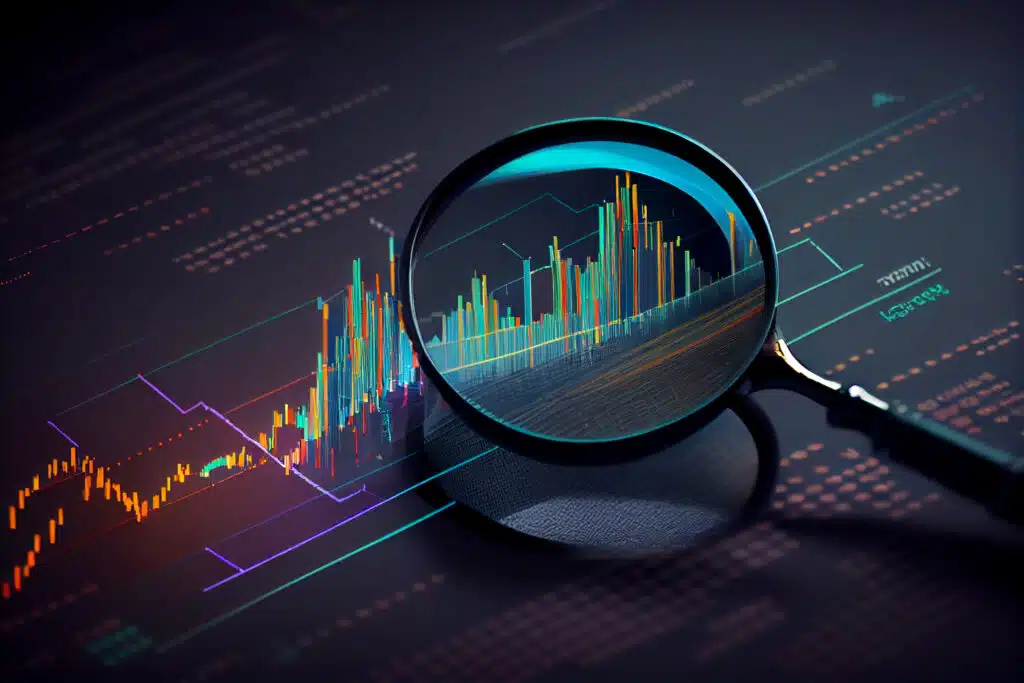
What’s left of human expertise?
It’s true that it has evolved considerably. The traders we often see in the movies, behind their computers or on the phone giving their orders, have generally been replaced by computers and algorithms. Today, most trading algorithms are developed by computer scientists. They are recruited by specialised companies, particularly in high-frequency trading. These profiles can provide a definite competitive advantage, as they have expertise in writing trading-specific codes and have developed expertise in optimising market access speed.
Is the market so predictable that it can do without human expertise?
While high-frequency trading requires an algorithm with the right strategies, it is true that the markets are well aware of them. It’s just that the time scale for putting them into practice is much shorter. Optimising this speed of access to the market is therefore crucial, because reaction time is essential: the operator with the shortest reaction time has an undeniable competitive advantage. These technical skills are very specific and generally concern people who have trained in computer science, for example. If there is one area of the economy where we can talk about Big Data, it is the financial markets. Every day, they generate an enormous amount of data in digital form. Every transaction, every order submission is a new piece of data. For high-frequency trading, it is therefore necessary to subscribe to data feeds that are sold by the exchanges. This requires highly advanced technical expertise and technological investments that are costly both in terms of human capital and computers.
Investors like you and me don’t have enough capital to access the opportunities these investors do when making these investments. The principle of high-frequency investing is to obtain modest profits on each transaction. These are very small profits, but because the transactions are repeated so many times, the profits are very large very quickly. If the investment is large, risk-taking is minimal, because the strategies are well known and reaction time is optimised to make the most of opportunities. The unpredictable part is relatively small. As a result, high-frequency trading firms have expanded rapidly over the last ten years. The profits that can be made are now much lower.
Can something go wrong with this algorithmic machine?
There are several famous cases of instability caused both by algorithms and to the detriment of algorithms, including the 2010 flash crash in the United States. The stock market crash lasted just over 30 minutes and was not directly due to high-frequency trading, but it did cause these operators to stop their algorithms, which probably amplified the crash. And then, as always with algorithms, there can be design flaws, bugs that were not anticipated. This was the case in 2012 for Knight Capital, a major US trading firm and one of the four major high-frequency trading operators. One day, one of their algorithms developed a bug and Knight Capital quickly recorded a loss of 440 million dollars and almost went bankrupt.
Are there other risk factors?
One area we still don’t talk about very much is the risk of intrusion by a platform or algorithmic operator. Ill-intentioned hacking, for example by terrorists, could seriously destabilise the market.
And will artificial intelligence play a role in the future?
We can expect the trading industry to use these tools one day. Some initial thought has already been given to this issue in a field other than the financial industry, namely online sales. Regulators, notably in the UK and the US, have shown that there is collusion between algorithms. Take Amazon’s pricing algorithms, for example: they are founded on artificial intelligence based on learning first, a self-learning process for repricing products. But they don’t just raise or lower prices depending on the context, they find ways to charge non-competitive prices. If they are not coded for this, they discover ways of making profits by creating implicit collusion.
Optimising the speed of access to the market is crucial, because reaction time is everything.
This has not yet become an issue for the financial markets, but it soon will, because it is part of the same question. In 2017, BlackRock, one of the largest American asset managers, began using artificial intelligence techniques to forecast returns and make portfolio allocations. The firm also relies on big data as part of its quantitative and traditional investment strategies. As for the investment bank JP Morgan, it has already announced the development of a forecasting tool based on artificial intelligence to anticipate the decisions of central banks.