Current developments in the medical applications of artificial intelligence (AI) have been described by many as a “revolution in medicine”. Yet even though advances in Machine Learning – and specifically Deep Learning – profoundly change how things are done, claims of a “revolution” are undoubtedly misleading.
Moreover, the idea, while it is appealing, suggests that medicine is bound to adhere to a paradigm shift oriented solely around the available technology. Whereas the way AI changes medicine could be driven by a more collective effort of those involved. If this were to be the case, the actual transformation would be an ongoing change for the decades to come rather of an instantaneous shift. As such, uptake of AI for medical purposes is unlikely to be the “revolution” we have been promised. Here is why.
#1 Changes in medical practise are slow
Precision medicine is a long-standing effort to leverage data for better treatments. Pioneered by Karl Pearson and Francis Galton in the late 19th Century, they were the first to collect data with the explicit objectives to statistically analyse them. Since then, amongst many efforts, during the second half of the 20th Century, the National Institute of Health (NIH) in the USA developed a wide range of statistical methods for precision medicine. The Human Genome Project in the 2000, and the development of more advanced hardware and software to support AI can therefore be considered as a continuity of these efforts.
AI comes as a continuity of previous technologies, rather than an immediate revolution.
Of the many examples of AI in medicine, radiology is another compelling example. The discipline developed with the Nobel-prize discovery of X‑rays by Röntgen in 1895. Radiography was the only technique for non-invasive medical imaging for almost 50 years. Over time, the gain of precision and ease of use made it one of the prime choices for diagnosis in many cases. For instance, surgeons used to diagnose appendicitis through touch alone, but now CT scans are the preferred choice. As with for precision medicine, AI comes as a continuity in those developments as did the introduction of previous technologies before it – rather than an immediate “revolution”.
#2 Hospitals are resistant to change
Radiology is one of – if not the – first medical disciplines in which the new generation of AI tools is being commercialised. The first scientific papers detailing proof-of-concepts using Deep Learning on radiographies were published in the early 2010s. And now, a decade later, the first tools are hitting the market. So, how did that happen? In part, the technology has matured but also changes were required on an administrative level.
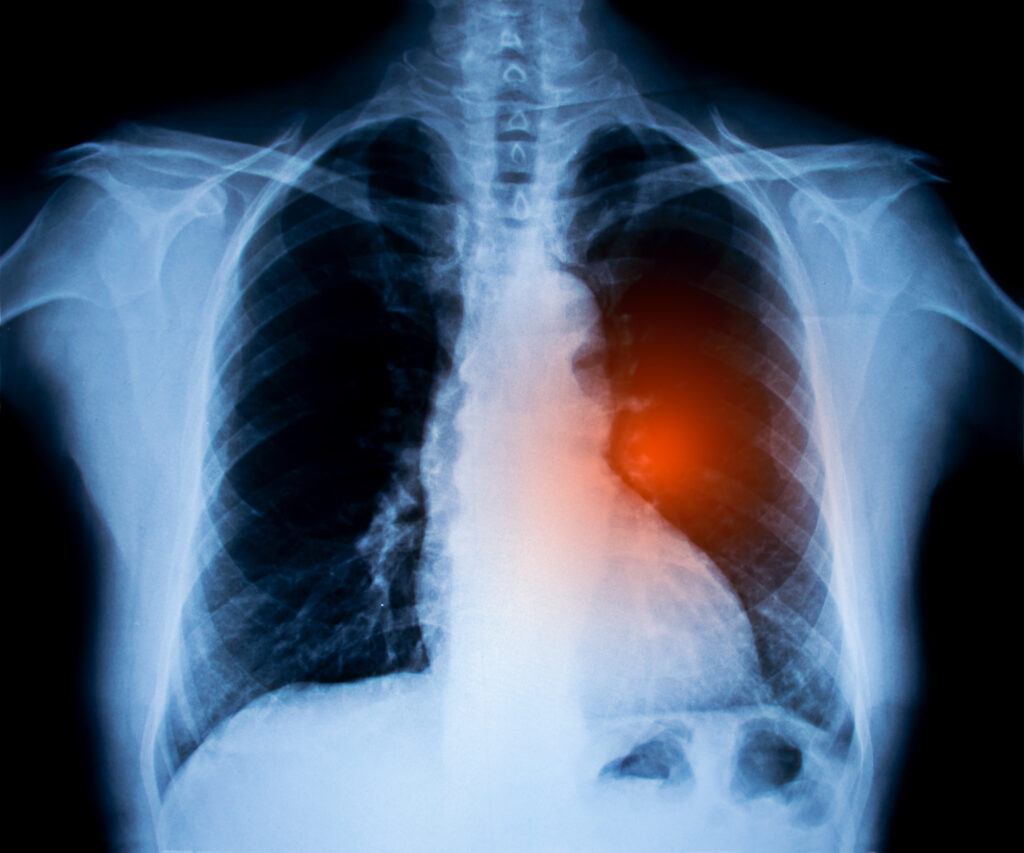
Even though some applications of the technology were developed five years ago, a span of time and investment was needed not only to build the AI but to obtain certification by regulatory institutions to use it. Now, authorisations are being delivered quicker since both parties learned how to prove the validity of AI applications – sometimes it is simply a matter of several months. Nevertheless, buyers and users still need to check the utility of the tool in their working context – i.e., patients’ needs and change of practise across hospitals.
Furthermore, hospitals need to find funds to pay for AI tools; since they amount to new practices, there is often no budget in place to pay for them. A hospital can take a year or more in administrative processes before buying AI and, although regulatory institutions may have validated the safety of a product, there remain few cases where those devices are reimbursed. The more novel, or the higher the “revolutionary” potential, the higher the barriers rise in the medical setting due to its conservative culture with regards to safety. The medical system pursues accuracy, an antagonist to the uncertainty of innovation.
#3 Data requirements are time-consuming
Rollout of medical AI applications is also limited by inherent characteristics of the technology. To start, data must be produced, and legal frameworks makes creating your own dataset very complicated – not to mention the time and money required. In many cases, developers go for “secondary use”. This term refers data not originally produced for the purpose with which it is being used such as diagnosis or administrative paperwork – not specifically for AI applications. However, this means that efforts are needed to clean the data, whilst still facing the many barriers: GDPR, authorisation accesses, monetisation, etc.
In addition, getting the dataset is only a milestone. Medical experts are needed to label data and help developers make sense of the results. Many iterations between data treatment and models are required before reaching a valid result. A rule of thumb estimates a proof of concept in AI is 80% data pre-treatment and 20% modelling. Add to that the proof-of- milestone stated above needed for regulatory bodies as well as users who must be convinced too.
Lastly, the scope of applications works best when performed tasks are narrow. The wider the scope, the more complicated and uncertain the development. For instance, current applications in radiology are often limited to detecting a specific area of interest in the body. Much of the time results there are false positives and complicated cases are seldom handled, such as breast-implants that often block mammography AI analysis.
#4 Uses of AI are still unclear
The early adoption of AI in radiology appears in a continuum of new practices. On one side, AI partially replaces the radiologist: some emergency services might use such tools to treat incoming patients when there is no radiologist on duty who could check results at a later stage. On the other, if AI serves for triage purposes it could be used as a second opinion to avoid a false negative. The difference in use could determine what is expected of the tool – precision, recall and other metrics would be calibrated accordingly.
Related issues, like responsibility, are still not formally addressed.
Such questions and related issues, like responsibility, are still not formally addressed. And how this pans out will ultimately affect AI development and use. Indeed, reliance on tools and automation is found to decrease expertise. If today senior radiologists can distinguish true from false in AI analysis, it is not the case for less-experienced ones. As young generations continue to rely on it, they might become more dependent and less critic about their results, which could be at the benefit of other skills.
Revolution or transformation?
Thinking there is a “revolution” in which we cannot influence the course of action is limiting. How AI applications develop may have more to do with current constraints on healthcare services – lack of personnel, funding, and resources – rather than optimal applications for medicine in general. Nevertheless, commissions are formed1 at the national and international level to regulate such issues, and perhaps other forms of collective decision could also be actioned, like the “communities of inquiry” developed by pragmatists as the cornerstone of democratic life2 .
The arguments provided above do not entirely discredit the possibility that a disruptive application for AI could appear that would “revolutionise” medical care. Yet, they do try to put the rate current developments into context and inscribe them in the decades-long process of the traditional innovation that happens in healthcare. And, more importantly, how the transition is not bound to evolve based solely on the technology – the slow rate of uptake in those innovations allow room for a collective action to how AI is used in healthcare.