Using epidemiology to combat cyberthreats
- The advent of digital technology has led to a proliferation of cyberthreats, and protecting against them is now a major challenge.
- Identifying potential risks, detecting threats, determining propagation mechanisms... the scope of cybersecurity is vast.
- To combat the spread of computer viruses, experts are drawing inspiration from epidemiology.
- In fact, computer viruses (like infectious viruses) have a unique signature that enables them to be identified.
- In particular, the SEIR model has been optimised to characterise the degree of sensitivity of computer hardware to contamination.
- This system seems particularly promising in terms of improving the security of IT systems, especially in the public sector.
The modern world has been built around digital technology, which is now central to the activities of businesses, organisations, and the state. At the same time, this new configuration has given rise to a proliferation of malicious actors. By taking advantage of this new opportunity, they are trying to achieve political, financial or even mafia-style aims. One of the most pressing challenges is to put in place IT security strategies to protect against these cyber threats.
Anticipating and monitoring in real-time to reduce risks
The natural reaction to a threat is often to flee or try to protect oneself. This is a natural mechanism aimed at warding off a threat that has just been detected. This reaction enables living things to survive in the face of predators and to protect themselves from their enemies. However, a more appropriate response in nature, as for any digital system, is to anticipate threats so as not to expose oneself to them1.
In the field of cybersecurity, it is therefore advisable to identify potential risks as part of a protection strategy. This is made possible by the EBIOS2 risk analysis approach recommended by ANSSI. Moreover, it is important to rely on real-time detection mechanisms such as “Dynamic Forecasting”3.
Drawing on epidemiology to combat the spread of computer viruses
During their research4, the researchers drew inspiration from epidemiological mechanisms to identify computer viruses. The idea is very similar to matching a criminal’s fingerprints or DNA: cybercriminals leave digital footprints too. Indeed, computer viruses have a signature that can be used to identify them (examples: MyDoom.A, Psyb0t, Chernobyl, Conficker, Cryptolocker…). This is often the mechanism used by antivirus software to detect them. For example, since August 2016, a piece of malware called Mirai, which focuses on connected objects, has been evolving to infect computer systems. This behaviour could be reminiscent of a virus such as COVID-19.
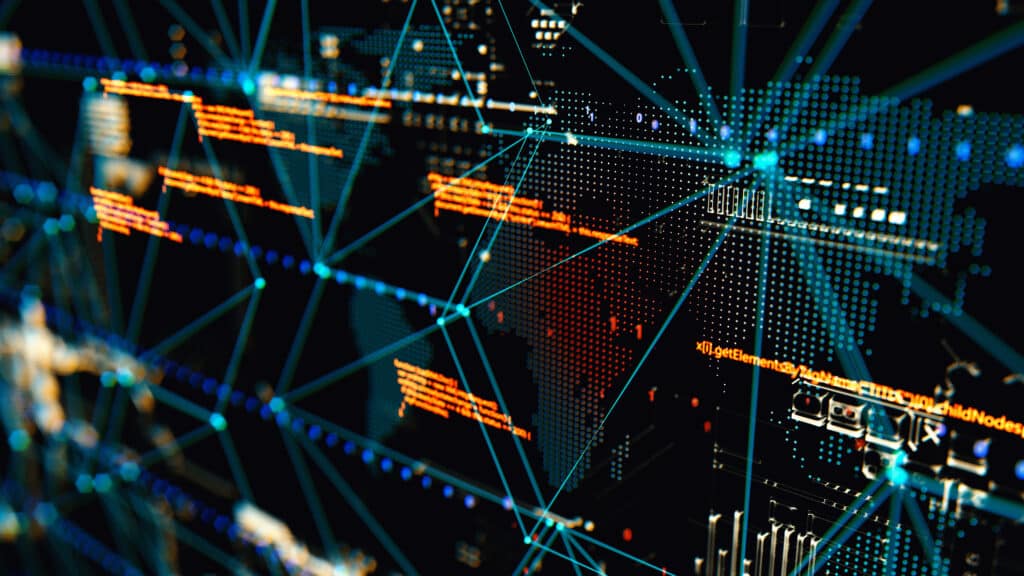
Our objective goes beyond simply identifying a virus. We want to determine its propagation mechanisms to better protect interconnected information systems. Government information systems may be particularly exposed to interactions with users, economic partners, and other government departments. As a result, a virus may not only be the result of an attack, but also of propagation from an infected partner.
The SEIR model
The SEIR model (Susceptible, Exposed, Infected and Recovered) is a well-established epidemiological tool. It is used in the fight against pandemics5, but also in the field of cyber security6. Adapted to our context, this model can be used to categorise computer hardware in different stages with regard to contamination by the virus under study. Hardware is considered “sensitive” if it is vulnerable to attack, “exposed” if it has been in contact with the virus, “infected” if it has been compromised, and “recovered” if it has been infected and has undergone remedial action to provide immunity against the virus.
Epidemiologists have long been familiar with this model7. In the context of digital environments, it corresponds to the situation where an ecosystem reaches a sufficient level of protection when it has been sufficiently exposed and protected by countermeasures. It then acquires a form of immunity that counteracts cyber-attacks based on the virus in question.
A multi-level collective immunity model with resource optimisation
In the course of our research, we identified limitations in the initial approaches to the SEIR model. While it provides a valuable framework, it does not take into account complexities such as the variation in system vulnerabilities or the evolution of attack types. Furthermore, the herd immunity model is conceptually powerful, but lacks a concrete method for achieving immunity. And this presents risks in the event of massive infection of the system.
We have therefore proposed a multi-level SEIR model with resource optimisation. This refined model incorporates two key aspects:
- Multi-level: it takes into account the different levels of security maturity and protection between different stakeholders, such as governments, private companies and individual users.
- Differentiated threats: it differentiates cyber threats according to their probability and potential impact.
The model uses parameters such as transmission rate, latency period and recovery rate to describe the propagation of cyber-attacks through different systems. It highlights the importance of fortifying cybersecurity throughout the ecosystem, as the weakest link represents the greatest risk.
Finally, a significant advantage of using this type of model is its ability to predict, at least in the short term, the behaviour of the virus. On the one hand, thresholds can be determined and, on the other, differential equations can be solved to predict behaviour and trigger automated alerts or responses via EDR (Endpoint Detection and Response) mechanisms.
Optimising the protection of critical systems with limited resources
A fundamental aspect of this new proposal is to focus on optimising resources in a context of limited financial resources. This model takes into account the limited budgets allocated to cybersecurity and focuses efforts on the major risks. This approach enables a specific strategy to be put in place for each system, focusing on the dimension that will reduce the overall infection as much as possible. Convex optimisation, a widely used mathematical method8, is recommended for solving this resource allocation problem.
The proposals put forward seem promising for improving the security of IT systems, particularly in the public sector. They are inspired by epidemiological methods, which are highly suitable for monitoring and combating the spread of viruses. Work is currently planned to test these proposals in real-life situations, implement them in operational systems and continue to improve the cybersecurity of critical systems.
Disclaimer: The content of this article is the sole responsibility of its authors and is not intended for any purpose other than academic information and research.