In 1957, a computer wrote a musical score for the first time. ILLIAC I – designed by Lejaren Hiller and Leonard Isaacson at the University of Illinois – composed a string quartet1. From then on, the promise of a computer program capable of generating music was rooted in reality. After all, music is all about structures, rules and mathematics. Nothing unknown to a computer program… except for one detail: creativity.
The fascinating thing about this suite is that it was composed by a computer, following a probabilistic model surprisingly similar to those used today2. Only, it was created according to rules established by a human composer, revised and then performed by an orchestra. The result: a rigid application of the rules, leaving little room for artistic innovation.
Today, technology has evolved radically: anyone can play at being a composer from their computer. And thanks to deep learning algorithms and the rise of generative AI, musical AI has taken an interesting turn. In order for a machine to really produce a musical work from scratch, it had to understand it, not imitate it. Therein lies the challenge of a scientific quest begun over twenty years ago: not to make machines compose, but to teach them how to listen. Recognising a style, classifying a work, analysing a musical structure…
Long before the explosion of AI-assisted music generation, researchers were already trying to get machines to hear music. Among them was Geoffroy Peeters, a professor at Télécom Paris and previously Research Director at IRCAM. His work on the subject could help us answer the question: can a machine truly understand music, even before it claims to create it?
Understanding music
“In the early 2000s, the international standardisation of the .mp3 format (MPEG‑1 Audio Layer III) led to the digitisation of music libraries (today’s streaming platforms), giving users access to a vast catalogue of music, and hence the need to classify and index each piece of music in it,” explains Geoffroy Peeters.
This gave rise to a new field of research: how to develop a music search engine? “These music analysis technologies are based on audio analysis and signal processing and were initially “human-driven”. Learning was based on human-input rules,” he adds. Music is not simply a series of random sounds, but a structure organised according using a rigorous grammar – sometimes as strong, or even stronger, than that of language. Since a style of music is determined by a certain type of chord, a certain tempo, a harmonic structure, and so on: “teaching these different rules to a machine didn’t seem all that complicated”.
“What defines Blues music, for example, is the repetition of a 12-bar grid based on the sequence of three specific chords,” elaborates the professor. These rules, which we know very well, will be encoded in a computer, so that it can classify the music according to genre.” That said, music is not only defined by its genre, but it can also convey a mood or be more suited to a particular context – be it for sports, or for meditation. In short, there are many elements whose rules are more diffuse than those determining genre.
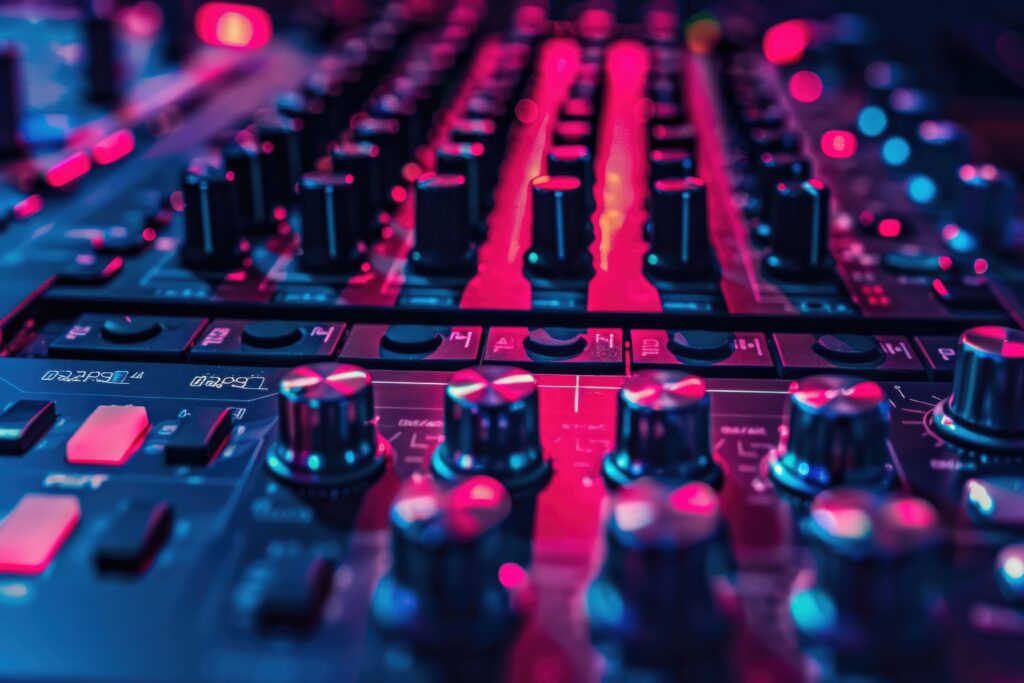
“In an attempt to address this complexity, Pandora Music, the largest music streaming platform in the U.S., created the ‘Musical Genome’ project, asking human beings to annotate over 1 million tracks based on 200 different criteria.” This colossal task has accumulated enough data to enable the development of so-called data-driven approaches (in which knowledge is learned by the machine from the analysis of data). Among machine learning techniques, deep learning algorithms quickly emerged as the most powerful, and in the 2010s enabled dazzling advances. “Rather than making human-driven models with complex mathematics, like signal processing, and manual decision rules, we can now learn everything completely automatically from data,” adds Geoffroy Peeters.
Over time, these trained models have enabled the development of classification and recommendation algorithms for online music platforms such as Deezer and Spotify.
Learning to listen
Deep learning will also bring about a paradigm shift. Whereas music used to be considered as a whole, it can now be analysed as a composite of elements. “Until 2010, we were unable to separate vocals, drums and bass from a mix in a clean – usable – way,” he points out. But if the voice could be extracted, the sung melody could be precisely recognised, characterised and more finely analysed. “Deep learning will make this possible by training systems that take a ‘mixed song’ as input with all the sources mixed (vocals, drums, bass, etc.), and then output the various sources demixed, or separated.” To train such a system, however, you need data – lots of it. In the early days, some training could be carried out with access, often limited, to demixed recordings from record companies. Until Spotify, with its huge catalogue of data, came up with a convincing source separation algorithm. This was followed by a host of new models, each more impressive than the last, including the French Spleeter model from Deezer, which is open source3, and Demucs from Meta-AI in Paris.
This individual analysis of each element that makes up a piece of music has turned AI training on its head. “All this has opened the door to many things, including generative AI developed today in music. For example, with the ability to separate the voice and analyse it in detail, it becomes entirely possible to re-contextualize it (reinserting Edith Piaf’s voice in the film “La Môme”, or John Lennon’s in the Beatles’ “Now and Then”), to modify it (pitch correction is widely used) to recreate it (the voice of General de Gaulle pronouncing the call of June 18th), but also to clone it. Recent events show just how far the latter use can go, with concerns in the world of film dubbing, the fear of “deepfakes”, but also a previously unreleased soundtrack with Drake and The Weeknd, which was nevertheless not sung by them.”
Becoming a composer
Early research in musical AI had well-defined objectives: to classify, analyse and segment music, and, why not, to assist the composer in his or her creation. But with the emergence of generative models, this work became the basis for a whole new approach: the generation of a piece of music (and therefore its audio signal) from nothing, or just a textual “prompt”. “The first player to position itself in music generation from scratch is OpenAI’s Jukebox,” notes Geoffroy Peeters. “In a way, they’ve recycled what they were doing for ChatGPT: using a Large-Language-Model (LLM), a so-called autoregressive model, trained to predict the next word, based on previous ones.”
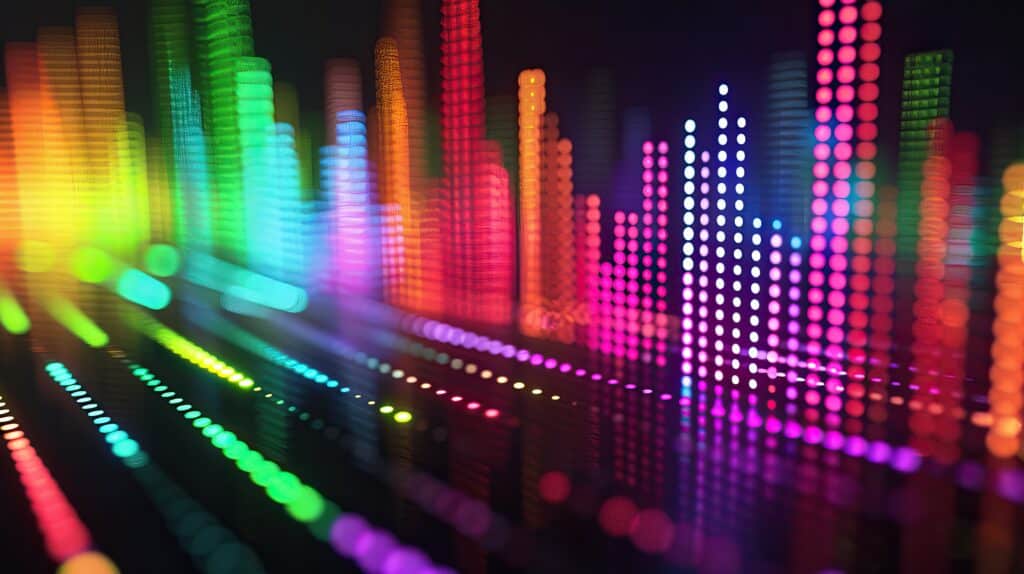
Transposing this principle to music is a major technical challenge. Unlike text, audio is not made up of distinct words that the AI can treat as tokens. “We had to translate the audio signal into a form that the model could understand,” he explains. “This is possible with quantised auto-encoders, which learn to project the signal into a quantized space, the space of “tokens”, and to reconstruct the audio signal from these “tokens”. All that remains is to model the temporal sequence of tokens in a piece of music, which is done using an LLM. The LLM is then used again to generate a new sequence of “tokens” (the most likely sequence), which are then converted into audio by the quantized auto-encoder’s decoder”.
Models with even more impressive results followed, such as Stable Audio from Stability AI. This type of model uses the principle of diffusion (popularised for the generation of very high-quality images, as in Midjourney or Stable Diffusion), but the idea remains the same: to transform the audio signal into quantised data readable by their diffusion model.
To provide a minimum of control over the resulting music generation requires “conditioning” of the generative models on text; this text is either a description of the audio signal (its genre, mood, instrumentation), or its lyrics. To achieve this, the training of the models will also take into account a text corresponding to a given music input. This is why the Suno model can be “prompted” with text. However, this is where the limits of their creative capacity and questions of intellectual property come into play. “These models suffer a lot from memorisation,” warns Geoffroy Peeters. “For example, by asking Suno in a prompt to make music accompanied by the lyrics of ‘Bohemian Rhapsody’ it ended up generating music very close to the original. This does pose copyright problems for the new music just created, because the rights to it belong to the human behind the prompt, and the music used for training the model, the rights to which they didn’t have.” [N.D.L.R.: Today, Suno refuses this type of generation, as it no longer complies with its terms of use].
“So, there’s a real need to turn these tools into models that generate new content, not just reproduce what they’ve learned,” concludes the professor. “Today’s models generate music, but do they create new music? Unlike audio synthesisers (which made it possible to create new sounds), music is an organisation of sounds (notes or otherwise) based on rules. Models are undoubtedly capable of understanding these rules, but are they capable of inventing new ones? Are they still at the stage of “stochastic parrots”, as is often said?”