Before tackling the issue of AI bias, it is important to understand how a machine learning algorithm works, but also what it means. Victor Berger, a post-doctoral fellow in artificial intelligence and machine learning at CEA-List, explains, “the basic assumption of most machine learning algorithms is that we have data that is supposedly a statistical representation of the problem we want to solve.”
Three main ways of learning
The simplest – technically speaking – most common way to teach a machine learning AI is called supervised learning. “For example, if you have a database full of animal pictures, a supervised algorithm will already know that a picture represents a dog, a cat, a chicken, etc., and it will know that for this input it should give a specific response in output. A classic example of this type of algorithm is language translators,” explains Victor Berger.
The second category of algorithms, unsupervised learning, is generally used when we do not have the solution to a problem: “to continue with the example of animals, an unsupervised learning algorithm will contain a database with the same photos as the previous one, without having precise instructions on how it should react in output with respect to a given input. Its aim is generally to identify statistical patterns within the dataset it is given for categorisation (or clustering) purposes.”
The whole problem lies in the data sets used to supervise the algorithms.
The third category of algorithms is reinforcement learning: “In the first two categories, the way the algorithm is coded allows it to direct itself and know how to improve. This component is absent in reinforcement learning, where the algorithm just knows whether it has completed its task correctly or not. It has no instructions about which directions to take to become better. In the end, it is the environment and its reaction to the algorithm’s decision making that will act as a guide,” Victor Berger explains.
In all three cases, the problem lies in the data sets used to supervise the algorithms. Victor Berger reminds us that “machine learning algorithms enable patterns to be identified. Therefore, the slightest bias hidden in a data set can bias the entire algorithm, which will find the biased pattern then exploit and amplify it.
Generalisation of data
For Lê Nguyên Hoang, a doctor in mathematics and co-founder of Tournesol, who popularises the subject of artificial intelligence, the hypothesis of the generalisation of data is omnipresent in the field of machine learning: “Questions relating to the quality of data are largely undervalued. Whether in the research world or in industry, it is the design of algorithms that takes centre stage. But very few people ask themselves whether generalising the past by training algorithms with historical databases that we don’t look at critically is really a viable project for society.”
To better understand how this can manifest itself, Victor Berger refers to a specific anecdote circulating in the machine learning community: “To avoid gender bias, a company using AI to sort CVs excluded information such as name and photos. But they realised that it had retained football as a relevant focus.” As careful as the company was, it provided its historical data without anticipating a pattern: the most recruited CVs in the past – those of men – were more likely to include the interest football. Far from combating the gender bias, the algorithm nurtured it. There are two solutions for dealing with this type of problem, “either humans are tasked with building more qualitative databases – but this requires a colossal amount of work – or algorithms are tasked with eliminating the biases already identified,” explains Victor Berger.
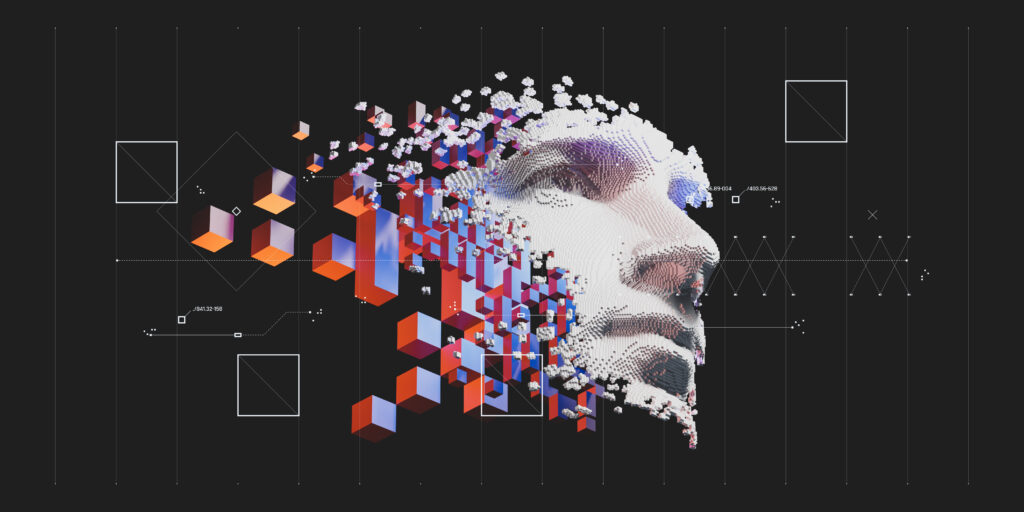
But this does not solve all problems. “If we take the example of content moderation, the labelling of data depends on the concept of freedom of expression that we defend, on what we consider to be or not to be a call to hatred or dangerous false information. Therefore, questions that do not have clear answers and where there will be disagreements. So, if the problem is not just a technical one, the same goes for the solutions,” says Lê Nguyên Hoang.
Feedback loops
There are also questions about the feedback loops which algorithms can cause. “What you have to bear in mind is that a machine learning algorithm is always prescriptive, because its aim is to achieve a specific objective: maximising presence on a platform, profit, click-through rate, etc.” points out Lê Nguyên Hoang.
Imagine an algorithm used by a community’s police force to predict in which neighbourhood there will be the most crime and aggression. Victor Berger argues that “what this algorithm is going to do is make a prediction based on historical police data that identifies the neighbourhoods where the most people have been arrested.” Here again, we fall back on the same flaw: the risk of generalising – or even amplifying – the past. Indeed, this prediction is not only descriptive, but it also leads to decision-making: increasing the number of police officers, increasing video surveillance, etc. Decisions that may lead to the reinforcement of a particular area of the city. Decisions that can lead to the reinforcement of an already tense climate.
The phenomena of radicalisation, sectarian movements and conspiracy circles can be amplified.
Similarly, on social media and entertainment platforms, recommendation algorithms are based on the user’s previous choices. Their objective is generally to keep the user’s attention for as long as possible. As a result, the phenomena of radicalisation, sectarian movements and conspiracy theories can be amplified. Lê Nguyên Hoang is working on solving this problem with the help of an algorithm, called Tournesol, whose database is built up in a collaborative manner1.
Questions of power
Artificial intelligence is therefore not just a field of scientific study or a technological field of application. It also has a great deal of power at stake. “It is very important to analyse and list the various social and ethical problems that can arise from these algorithms, from their training to their design and deployment,” warns Giada Pistilli, a philosophy researcher and senior ethicist at Hugging Face.
What exactly are these problems? The philosophy researcher explains that they can be found at all levels of the AI development chain. “There can be ethical problems that emerge as soon as a model is trained because of the data issue: can the data lead to stereotyping? What are the consequences of the absence of certain data? Has the data used, such as private images and intellectual property, been subject to consent before being used as a training dataset for the model?”
But this is far from being the only problematic link in the chain. “During development and deployment, questions of governance arise. Who owns the model, who designs it and for what purpose? There is also the question of the need for certain models in the light of climate change. Running these models consumes a great deal of energy. In fact, this highlights the fact that only powerful companies have the resources to use them,” warns the researcher.
We can make AI a real empowerment tool that communities could take ownership of.
Fortunately, the picture is not all black. Artificial intelligence can be used as a tool for empowerment. Giada Pistilli is a member of BigScience, a collaborative project involving thousands of academics that aims to develop an open access language model. According to her, such projects can make AI robustly beneficial. “By developing AI that is specialised on a single task, it can be made more auditable, participatory, and tailored to the community that will use it. By educating users about these new technologies and integrating them into the project of building databases, we can make AI a real empowerment tool that communities can take ownership of.”
Will we be able to rise to these multiple challenges? The question remains.