Reproducibility, meaning other scientists can obtain a comparable response by recreating the same conditions as an original study, is imperative to the scientific method. In short, the results of an experiment should be the same whoever carries out the procedure. And for most cases, this is true. However, across various disciplines in the social sciences but also in biomedical research, certain scientific studies have failed to replicate when carried out later by other scientists – questioning the results of the original studies123.
Known as the “replication crisis”, the problem concerns more than a few single studies published in low-tier journals. Rather, the crisis is systematic, affecting as many a third of social science studies – involving even the most prestigious journals such as Science or Nature4. Affected studies include various well-known phenomena, many of which have found their way into the public. Amongst them are widely spread concepts such as stereotype threat5, implicit bias6, or social priming7. Those are just three of the most famous findings facing serious criticisms, so much so that they might not survive further methodological scrutiny. But how did we get into this crisis, and what can we do about it?
P‑hacking, HARKing and publication bias
Specifically, two of the most common “bad research” practices responsible for non-replicable results are due to statistical manipulation: p‑hacking and HARKing. In the former, researchers tweak their research design slightly until a non-significant result turns significant – essentially turning something from a negative result to a positive one. For example, after failing to find any effect in their experiment, the researchers might change the way variables are measured, exclude a few outliers that were not excluded before, or stepwise collect a few more participants, checking each time whether the results have become significant. All of these practices increase the chance that the researchers will find an effect even if the effect actually does not exist.
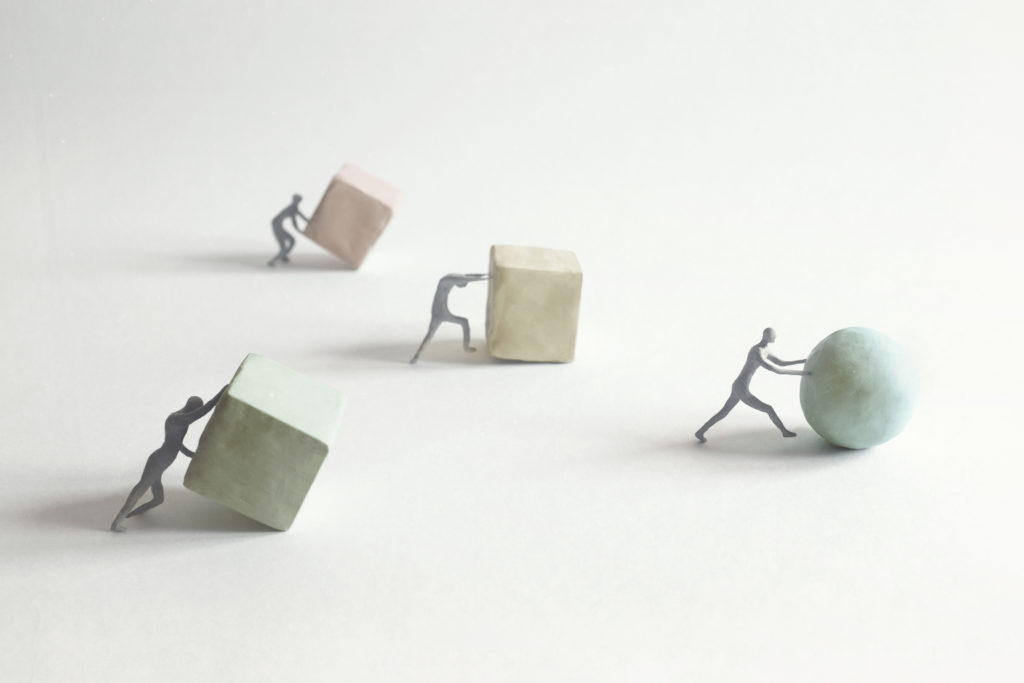
Similarly, in HARKing (hypothesising after results are known), researchers randomly find an effect between two variables and then hypothesise that this is what they expected all along. FYI: a hypothesis is made before an experiment is carried out – not retroactively. In today’s age of big data, it’s not hard to see why this is a bad idea. In a large dataset containing hundreds of variables, some of these variables will be correlated with each other just by chance. Claiming that you only expected an effect for these correlated variables even though you ran correlations for all variables gives a distorted view of the actual data.
In the academic world, publications are the gold standard of success, but scientific research is much harder to publish if no significant results are found. As such there is a publication bias. Hence, if you want to have a thriving career in science, you had better find an effect! Of course, this doesn’t fully explain why significant results are so important. After all, the results of an experiment do not tell us anything about the quality of the methods used. If a study does not find the effect, it might simply be that that the effect does not exist. Yet, scientific journals nevertheless often refuse to accept non-significant results for publication, because non-significant results do not prove the absence of an effect to the same extent as significant results proof the existence of an effect.
In standard social science research, the highest acceptable false positive rate is 5%, while the highest acceptable false negative rate is 20%. In other words, many scientific studies are not adequately powered – meaning they do not have enough participants to decrease the false negative rate to an adequate level. As a consequence, journals may reject studies with non-significant results on the grounds that the study could have found the effect if the sample size had been larger.
Pressure for a “scoop”
All of the aforementioned questionable research practices – p‑hacking, HARKing (which arguably is a subtype of p‑hacking), publication bias, and underpowered studies – are well-known issues by now, but the problems of the replication crisis run deeper. One of the reasons why many classic studies were found to be non-replicable only decades after the original studies were conducted is that there is little incentive to do replication studies. Academic careers thrive on pursuing novel ideas, as journals are likely to dismiss replication research due to its lack of originality. Hence, there is not sufficient replication research that would either red-flag original studies if the results are not replicated or provide more certainty for those results that are successfully replicated.
A related consequence of the lack of replication of older studies is that it is difficult to estimate the extent of the reproducibility crisis.
A related consequence of the lack of replication research is that it’s hard to estimate the magnitude of the replication crisis. Aside from social science and biomedical research: which other disciplines are affected? And to what extent? Until replication research becomes common practice, we can only speculate about the answers to these questions.
While it’s hard to think of a suitable way to integrate regular replication studies into the current research system, registered reports could provide a solution to all four of the bad research practices mentioned here. Unlike normal journal articles, registered reports are accepted for publication before data is collected. Hence, the problem of publication bias is solved as the results cannot influence the journal’s decision whether or not the study will be published. P‑hacking and HARKing are also unlikely to occur since the researchers have to specify in advance which and how hypotheses will be tested, and any deviation from the research plan needs extraordinary justification. Finally, registered reports are generally more adequately powered than normal journal articles, as the methods (including the intended sample size) are reviewed before the study is conducted.
Would a more replicable science lead to more public trust in scientific findings? We don’t know, but it’s likely. If the scientific community accepts that certain research findings are indeed dubious and attempts to improve on these shortcomings, maybe science sceptics will be less reluctant to accept research results that are actually robust. We certainly still have a long way to go until the crisis fades, but fostering methodological skills, adopting registered reports as a publication model, and incentivising replication research are promising first steps in the right direction.