How does one measure the intelligence of an artificial intelligence?
It isn’t easy because the term “artificial intelligence” is used by the general public to refer to objects developed in this research field, such as software equipped with a learning system. In fact, artificial intelligence is not a thing. Rather it is a field of study which tries to model functions of the human mind, like memory, reasoning, learning or language. We therefore cannot measure intelligence.
Moreover, the notion of ‘intelligence’ makes no sense generally speaking. For example, it’s impossible to say that an earthworm is more stupid than a human. Instead, each living being has behavioral and morphological characteristics resulting from an evolutionary process related to its particular environment. Earthworms can find food in the ground. And, in their own ecosystem, human beings have social interactions – linguistic or cultural – with others. An earthworm wouldn’t know what to do in our ecosystem and a human wouldn’t do any better in soil.
Technologies, too, are developed in very specific contexts. It cannot be said that smartphone voice recognition systems are stupid because they don’t understand the meaning of the sentences that they transcribe. They were not trained to that end, it’s not part of their ‘ecosystem’.
The software you mention transcribes and learns, could it also understand?
Fundamentally, the meaning we associate with a sentence is personified, it is interpreted based on the sensory and motor experiences of our body in its environment. If a machine does not have access to a body to physically interact with our world, it stands no chance of interpreting sentences like we do.
However, we can train language models with large text databases. Machines can then detect statistical patterns and do astonishing tasks, such as answering a simple question, by predicting the structures of sentences according to a given context. These tools are very useful in the world of industry, for human-machine interface, when machines must interpret directives based on the context. In order to do this, unlike humans, they don’t necessarily need to understand sentences.
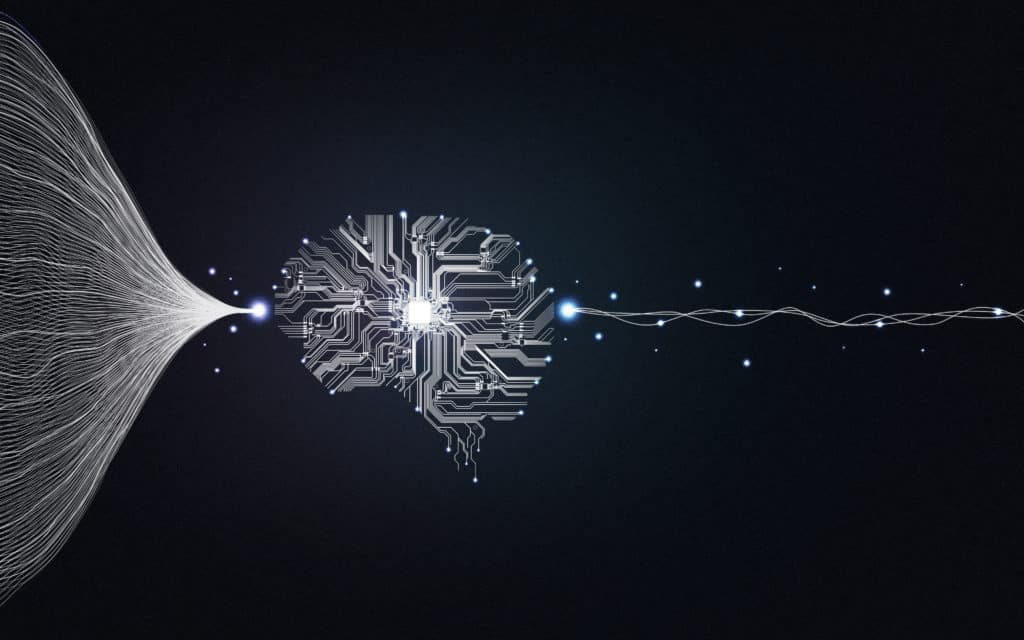
In your research you state that, in humans, part of learning is driven by curiosity. Can it be applied to software?
This issue is at the heart of my team’s research. We study the mechanisms of curiosity, or what psychologists call “intrinsic motivation”. It allows living beings to undertake independent learning. We develop algorithmic models of curiosity to highlight the mechanisms involved, such as spontaneous exploration. This plays a fundamental role in the sensory, cognitive and motor development of humans.
We then test our theories with volunteers or machines. In doing so, we discovered that to efficiently explore its environment, a robot must pick the areas where it makes the most progress, meaning those in which the gap between prediction and reality tends to decrease. For example, it is in its interest to play with an object with which he tends to make progress rather than another that he immediately masters or, on the contrary, that he cannot use at all. We showed that, in theory, this strategy is efficient for robots. The question remains open as to whether humans use this measure to make progress and to guide the exploration of their surroundings.
But could this measure of progress explain why humans tend to prefer activities that they are able to learn easily?
Yes. The mechanism of exploration according to progress leads to a “snowball” effect: when exploring an activity, initiated randomly or other contingent factors, we develop knowledge or skills which will make similar types of activities easier to learn. This encourages the individual to pursue that course of action; also associated with the pleasure response in the brain upon exploring new activities.
This fundamental hypothesis explains the diversity of learning trajectories found in different people. To confirm that, we compared the behavior of adult volunteers to that predicted by our computational model. These analyses showed that learning progress and performance for each task are measures used by humans to guide their exploration. Both work in different ways: the combination of these differences and the snowball effect mentioned above supports the idea that there is a diversity in learning pathways, which explains differences between individuals.
Does this model improve machines?
Our theories can sometimes be implemented in machines to make them more adaptable. But the exploratory behavior of humans is not necessarily the most optimal choice. For example, other curiosity mechanisms are better suited for robots destined to autonomously explore the ocean floor or the surface of Mars – if only to prevent these machines from making dangerous choices as much as possible.
Can these tools also help humans to learn better?
Yes, there are indeed applications in the field of education. We designed software that created custom exercise sequences for students in mathematics. The objective is to adapt a series to each child that will optimise both his/her learning and motivation. We know that the latter is an important factor in academic failure. Motivational aspects prompt students to persevere and try harder. Using curiosity models, we developed algorithms that interact with each child individually to offer a motivational series of exercises according to the child’s profile.
In a previous project named Kidlearn we showed that, on average, a greater diversity of students made more progress thanks to our software’s proposals than with a teaching expert. A figure that includes children with a range of difficulties or abilities, too. This benefit was associated with a higher degree of intrinsic motivation. We are now working with a consortium of industrials in the field of education technology (edTech) in order to transfer this approach into a digital educational system intended to be used on a large scale in primary schools in France (Adaptiv’Maths project). My colleague Hélène Sauzéon even showed that this system can facilitate learning for children suffering from developmental disorders such as autism.