AI and machine learning are already used today to help diagnose patients. How can they be useful?
Mounim El Yacoubi. First of all, it must be stressed that diagnosis is not just a matter of sorting out patients. There is no clear line between that which is “normal” and that which is “pathological”. This is why doctors remain in charge of their diagnoses, and why machine learning solutions only exist as aids intended not to replace them but to help them prioritise patients.
Hence, today, machine learning has a contribution to make in medical diagnoses, particularly concerning the detection of anomalies in MRIs. This type of method is based on supervised learning using millions of images, in which the systems are able to detect anomalies, with very high classification rates – sometimes in even finer detail than those of doctors.
So, you are saying that AI can be used to go beyond current health testing?
Yes, it can. Traditional diagnostic methods, which rely on blood tests, medical imaging or the measurement of other biological parameters, try to identify an anomaly or the characteristic symptoms of a pathology. They work fairly well but are not perfect because they are often invasive and costly in terms of equipment and personnel. Patients also have to come to the hospital or medical laboratory. For all these reasons, diagnostic tools based on machine learning, on data from inexpensive and non-invasive sensors, are of interest to the medical community.
You are working on techniques using data that goes beyond traditional medical testing?
We work on so-called “ecological data”, such as handwriting, gait or voice. For Parkinson’s disease, we are conducting a European research project in collaboration with the Institut du Cerveau et de la Moelle épinière in France. The aim is to be able to detect abnormalities in a patient’s voice and facial expressions – that are characteristics of the disease – during a simple video call.
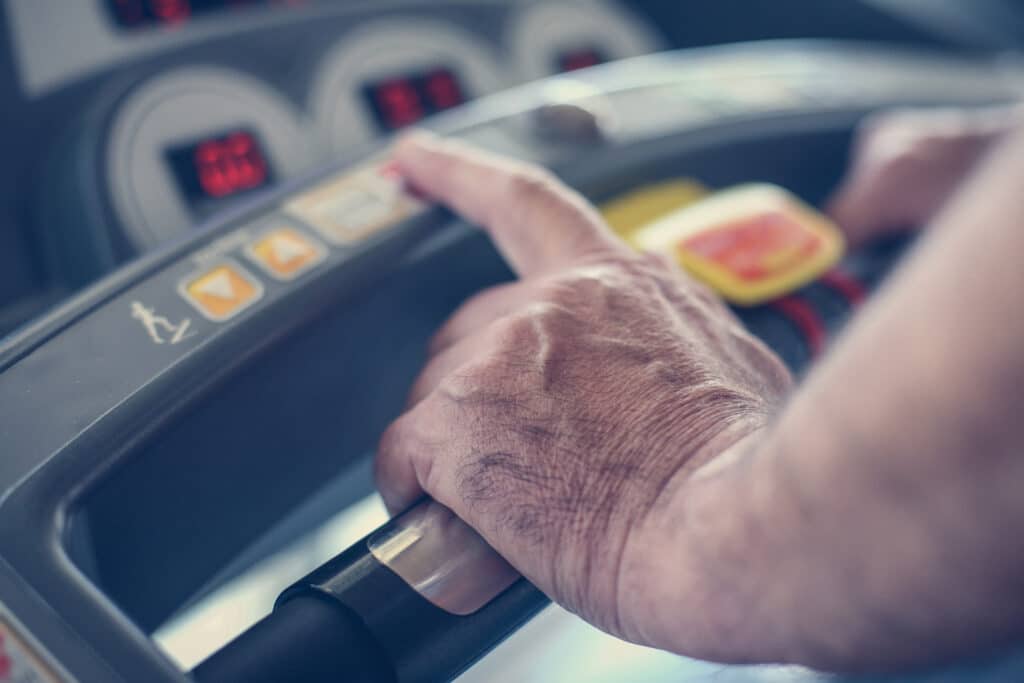
People suffering from this neurodegenerative disorder generally show hypomimia, i.e., a reduction in the amplitude of expressive movements, or voice alterations. We are developing a machine learning method to automatically detect these signals, and we aim to compare these results with MRI data or other clinical indicators. We hope that our approach can help to better characterise patients and stratify the disease; meaning that we will identify criteria for detecting groups of Parkinson’s patients with different behaviours, who could therefore be treated by doctors with different treatments and therapies.
With a tool like this, a first diagnostic step could be made without even needing to bring the patient into the medical centre!
Will they be able to use data that is currently imperceptible to doctors?
In theory, the doctor could detect these signs, but in practice it is very complicated, because you would have to compare how facial expressions evolved over several months. We developed a similar approach for Alzheimer’s disease, in collaboration with the Broca Hospital in Paris. The aim was to identify the deterioration in handwriting, voice and walking attributable to the disease. For this work on neurodegenerative diseases, the challenge is to reconcile specificity and sensitivity. We want to be able to identify patients with early forms without confusing them with other neurological disorders, such as mild cognitive impairment or other pathologies. It’s very tricky.
Can connected devices help you deploy these approaches?
For type‑2 diabetes, we use connected blood glucose sensors. They allow us to read blood glucose levels continuously; we don’t need to ask patients to prick themselves and collect measurements 24-hours a day. We combine this data with information on meals and insulin intake, which the patient can give us via a diabetes tracking application on a smartphone, and their physical activity, which is recorded via a connected bracelet. By combining this information, we can predict the blood sugar level.
The aim was to identify handwriting, voice and walking impairments caused by the disease
This is a real challenge because each person has his or her own metabolism, his or her own genetics… We have therefore created personalised models based on ‘sequential deep learning’ models. This work was the subject of a thesis by Maxime de Bois, which I co-directed with Mehdi Ammi from the University of Paris-Saclay. Maxime developed his technique on a synthetic patient base, validated by the FDA, the American regulatory authority. He then tested it on 6 patients in collaboration with the Revesdiab network.
Did you encounter any difficulties?
Yes, several, but we were able to resolve them. To overcome the lack of data, we use a transfer learning method, which allows us to pre-train the model from other patients, ensuring that it generates the most general parameters possible, and therefore the most adaptable to a new patient. To improve the acceptability of the system to doctors, we have taken into account the differences in predictions in our choice of metrics.
To explain how our model works, we integrated layers into our deep neural network (the learning method) to estimate the weight of each variable over time. For each prediction, we are thus able to indicate, at each point in time, which variable (blood sugar, food or insulin) was decisive. This is also a very interesting aspect because the doctors themselves do not know which parameter is greater at a given moment.
Is this your only project with connected objects?
No, we also have a project to improve the diagnosis of cardiac arrhythmia using a connected bracelet that measures arterial stiffness. Here too, we will compare our results with those obtained with electrocardiograms.
Do you think that, in the future, our connected fridge will be able to alert us to a risk of depressive behaviour?
It is indeed a good object to spot changes in habits… One can imagine that these data could be correlated with those of a smartphone or with the nature and activities on the websites visited. This will raise a major data protection issue. Will we allow our doctor to consult the analyses from our fridge? Will our search engine or social networks warn us if our behaviour changes in a dangerous way? One imagines that people with chronic pathologies and who experience phase changes, such as diabetics or sufferers of bipolar disorder, would be more likely to give informed consent to this type of approach.
For further information:
- DIGIPD : Validating DIGItal biomarkers for better personalized treatment of Parkinson’s Disease, https://www.erapermed.eu/wp-content/uploads/2021/01/Newsletter-ERA-PerMed_final.pdf, 2021.
- Maxime De Bois, Mounim A. El-Yacoubi, Mehdi Ammi, “Adversarial multi-source transfer learning in healthcare: Application to glucose prediction for diabetic people,” Computer Methods Programs Biomedicine, 199: 105874 (2021).
- Maxime De Bois, Mounim A. El-Yacoubi, Mehdi Ammi, “Enhancing the Interpretability of Deep Models in Heathcare Through Attention: Application to Glucose Forecasting for Diabetic People,” International Journal of Pattern Recognition and Artificial Intelligence, to appear, 2021.
- Mounîm A. El-Yacoubi, Sonia Garcia-Salicetti, Christian Kahindo, Anne-Sophie Rigaud, and Victoria Cristancho-Lacroix, « From aging to early-stage Alzheimer’s: Uncovering handwriting multimodal behaviors by semi-supervised learning and sequential representation learning, » Pattern Recognition, Vol. 86, pp. 112–133, 2/2019.
- Saeideh Mirzaei, Mounim El Yacoubi, Sonia Garcia-Salicetti, Jerome Boudy, C Kahindo, V Cristancho-Lacroix, Hélène Kerhervé, A‑S Rigaud, “Two-stage feature selection of voice parameters for early Alzheimer’s disease prediction,” IRBM, Vol. 39, No. 6, pp. 430–435, 2018.